Abstract
A database is simply a systematic collection of information organized and stored in computer systems, for example, Microsoft Access or Oracle database. It is controlled by a database management system (DBMS) and makes data management easy to access, modify, control and update. There are various database types, e.g., centralized database, distributed database, cloud database, and network database, to name a few. This paper will tackle database systems and security by identifying a problem of interest and finding credible solutions.
Furthermore, I will dwell on the evolution of data and its storing systems, determine how people refer to large amounts of data and how it affects information processing. In the second part, I will discuss how big DATA and its technologies affect the population, emphasizing scientific work in the digital arena. The primary focus will investigate big data using course management systems (CMS) like Moodle and Canvas. Study them comparatively to establish similarities and differences in big data processing. This will help determine which platform is better for storage and transmitting massive information.
Evolution of Big Data and Data Storage Systems
Database security refers to the range of tools, controls, and measures designed to protect and preserve the database’s integrity, confidentiality, and availability. They are put in place to cover sensitive data, DBMS, physical and virtual servers, network infrastructure, and associated applications (Brumm, 2019). Data security is vital for businesses as they are considered valuable assets. It ensures that information is safe and does not fall into the wrong hands and potentially bring down the business.
Data has evolved over the years from simple to complex forms. Before the industrialization era and the development of computing devices, data was collected manually. People had to go out, do fieldwork and impose patterns on their findings. This was time-consuming, tiresome, and required a much-skilled workforce to categorize it accordingly. However, with the development of computers and the digitalization of devices, machines can now collect data solely. Computers collect data, sorts it out, processes it, and stores it in this day and age in simplified formats (Periasamy & Raj, 2016). Many companies are trying to harness the value of information. Figuring out how much data is enough, which data is relevant, and how to measure return on investment. This is particularly important in the e-commerce sector, which has seen a growth in transactions.
The world is in the face of user-generated content. Social media has enhanced both structured and unstructured information, thus necessitating new analytics to understand their value. Traditionally, platforms were used to analyze the workflow of an enterprise, from the HR department to financial management (Periasamy & Raj, 2016). They were used to make modifications to the workflow and improve the organization. But with digitalization, the new world is end-user targeted, for example, commercial travel. Before, flights would only list prices from destination A to B. But with digitalization and the evolution of data, you get to choose a budget and have the intelligence to suggest better destinations. Scientists are also making incredible strides in the machine intelligence department with a key focus on enhancing artificial intelligence.
Evolution of Big Data Storage Systems
Big chunks of data are processed in every industry daily; thus, data storage needs have increased, creating a continuous demand for storage capacity. Data storage began after the floppy disk drive (FDD) invention by Alan Shugart at IBM (Martins & Soofastaei, 2020). In the 1990s, fierce competition among companies to produce smaller HDD was characterized by bigger capacity, smaller sizes, but cheaper forms. In recent years, data storage has evolved from object storage to solid-state drivers to what we presently have; cloud-based technology and flash storage. These changes have made the market more dynamic.
Cloud-based technology benefits converged infrastructure that groups multiple IT components into a single, optimized computing package. It solves IT skills as it centralizes management of resources, increases its utilization, and lowers cost. Flash storage is solid-state storage that uses electricity in surface-mounted chips and addresses the energy drain problem caused by hard drives. It has seen mass adoption by data management companies even though it is slightly expensive (Martins & Soofastaei, 2020). It is poised that the industry will advance even further from one-time storage uses to multi-purpose use to store the ever-expanding amount of data consumed daily by big data management.
Big Data Technologies and its Effects
In layman’s terms, Big Data is huge data. It combines structured, semi-structured, and unstructured data that contains greater variety with increasing volumes and has more velocity (Brumm, 2019). Examples include jet engines and the stock exchange market. Big Data is collected on networks, majorly from three primary sources: social data, transactional data, and machine data. However, companies are not restricted to just these three. Other ways in which big data can be used include using loyalty cards, gameplay purchases, satellite imagery like google earth and maps, and even personal mailboxes.
Real-time data is produced from streaming platforms like YouTube, Twitch, Netflix, Skype, Zoom. These are easily accessible from the internet and mobile networks (Brumm, 2019). Organizations mine this information and use it in machine learning projects, analytical applications, and predictive modeling. Some of its applications are in the E-health sector to personalize health services, transportation, and logistics to track shipments and improve services (Oussous et al., 2017). The Smart Grid manages real-time national electronic power consumption while monitoring operations.
Big data affects the world economy and its population in areas such as labor and capital. These effects can be positive or negative from different viewpoints. On the positive side, Big Data helps group complex data with large volumes to diverse varieties and links many data sets together. Researchers can also create simplified models that are easily readable and understandable to predict the economy better. Big data helps produce new goods and services that are customer-based, thus giving businesses an edge. With Big Data, companies customize their goods and services to the customers’ needs taking into account their preferences and styles. With this, organizations can target their audience directly and increase their profit margin tremendously.
McKinsey Global Institute reports that value growth of up to $3 trillion could be generated from Big Data every year (Kennedy, 2020). This would help improve infrastructure, ease traffic congestion, and ensure low prices of goods and services beneficial to the customers at large. Furthermore, businesses and governments enjoy faster innovation through shorter research and development cycle. With proper analysis of Big Data, organizations can increase specialization to improve areas doing poorly in their respective sectors. Governments’ open data policies would help combat corruption, improve workplace conditions, increase revenue, and improve foreign trade. Lastly, with Big Data, people enjoy newscasting, developing inferential software, and creating advanced algorithms for correlations. This means the public receives real-time forecasting of events, project outcomes are assessed to date patterns, and better understand the world.
In contrast, Big Data also has some negative impacts on the population. One significant adverse impact is privacy concerns. Businesses such as Google and Facebook surveil their customer’s lives as they try to get information (Herold, 2014). They then sell this information to organizations to market their products. This breaches their privacy and exposes their identities to the public. For example, consider a teenager using drugs. If this information was to be sold, they may gain access to more harmful drugs and worsen their situation. In addition, few (if any) legal protections exist to protect individuals if casualties arise. Secondly, Big Data is a relatively new subject that requires a high degree of fine-tuning for optimal performance and may not offer unique solutions. Also, Big data analytics are not 100% accurate (Herold, 2014). The algorithms may be flawed or may contain inaccurate data, thus providing false predictions. This is further enhanced by increasing data sets without considering validation in the analysis. The result will have misdiagnosed conclusions which may harm the company’s image.
Collecting, analyzing, and storing Big Data may prove costly at the expense of the public. Companies will invest heavily in technology and human skills to analyze such data while still trying to attain profit margins (Kalema & Mokgadi, 2017). The cost will then fall to the consumers, who will have to pay more for the goods and services. Behavioral profiling may bring price discrimination as it allows advertisers to offer goods at different prices in ways that maximize revenue extracted from each transaction. For example, a 2012 Wall Street Journal article found that major companies like Depot, Rosetta Stone, Staples, and Home Depot systematically used a physical location to display different online prices to customers (Mills, 2019). This will generally raise the prices and often hurt low-income households.
Seedy companies use behavioral profiling for financial scams, which are offered to vulnerable populations in financial crises. Racial profiling discriminates against black people in the USA. Companies like Wells Fargo collect zip codes of browsers and direct those buyers to similar racial neighborhoods. From 2004 to 2009, Wells Fargo illegally steered an estimated 30,000 Hispanic and black lenders into costly mortgages and charged them higher fees than their white counterparts (Kröger et al., 2021). I believe that it is up to the federal government to take action to protect vulnerable consumers through strengthening user control of personal data and increasing regulators. The government can also enforce structural changes in the market to increase competition where there are many companies. Finally, they can directly regulate Big Data platforms to prohibit harmful practices like price discrimination by baring data platforms.
In summary, as more of the economy moves online, society must get ahead of the curve and handle information. It is not solely up to the government to find solutions to the problems. A few tweaks would go a long way in improving Big Data’s management and enhancing its acceptability in all sectors. Big Data is here to stay, and we might as well learn to use it to our advantage. However, there is still room for improvement.
Moodle’s Scientific Framework
Moodle is an online open-source, part learning management system (LMS) and part course management system (CMS) developed by Martin Dougiamas to help educators create courses online focusing on content interaction and is continually evolving. It is used for blended learning, flipped classrooms, distance education, and other e-learning projects in workplaces and schools (Mogaji, 2018).
It is coordinated by Moodle HQ supported by a network of eight-four Moodle Partner service companies worldwide. Moodle has adopted several e-learning standards like Learning Tools Interoperability (LTI), a standard way of integrating learning practices with educational platforms (Mogaji, 2018). It has also adopted the AICC HACP in the 2.1 version and Sharable Content Object Reference Model (SCORM) that defines communications between client-side content and a server-side LMS. Moodle shares range 50% in Europe, Oceania, and Latin America (Hill, 2017). Moodle e-learning platform offers the possibility of creating courses, accessing the content by students, submitting assignments, and having report forms.
There are functionalities, modules, and resources. The resources are in digital format and include PDF documents, PowerPoint presentations, Word documents. Modules like Forums, Quizzes, and charts enable the interaction of teachers and students (Muhtar, 2017). Moodle has a MySQL database, and the size is in gigabytes. To facilitate standard procedures, Moodle uses processing tools to extract knowledge. One such tool is the Cloudera Hadoop which distributes storage and processing of large data sets. The critical components of the Hadoop framework are the HDFS (Hadoop Distributed File System) which store large amounts of data, and the Map-Reduce that represents the processing layer designed to handle large volumes by dividing the work into independent tasks.
HDFS provides querying and analysis of data stored through Hive, a warehouse infrastructure software built on Hadoop. Hive chooses servers to store the metadata of tables and columns, their database types, and HDFS mapping. Hive also provides an SQL-like language called HiveQL for querying structured data because the Map-Reduce framework is shallow and requires writing custom programs (Liao et al., 2016). Hadoop cluster also contains Impala, which is a massively parallel processing SQL query engine. In terms of execution time, Impala is faster than Hive because it implements distributed architecture based on daemon processes responsible for all aspects of query execution. This ‘Hadoop in the cloud’ paradigm is identical to a server that is accessed remotely (Jlassi & Martineau, 2016). Running Hadoop in the cloud benefits flexibility, low risks, cloud provider features, efficiency, and reduced cost.
Cloudera can deploy Cloudera distribution, including Apache Hadoop (CDH), in the public environment, working hand in hand with products like data engineering, operational database, and analytical database. Data engineering provides CDH components for fast and cheap data storage like Spark (Lindell, 2020). The analytical database provides CDH devices for discovering, analyzing, and understanding data, including Impala and navigator. In contrast, an operational database provides building data-driven applications comprising of HBase and Spark Streaming. The process of data preprocessing is considered crucial in the data analytics process (Akçapınar & Bayazit, 2019). The process moves from obtaining information in the Moodle database to depersonalizing. It then moves to Hadoop HiveQL for querying, and later on, it uses Rapid miner or WEKA to cluster the database (Bante & Rajeswari, 2017). Finally, reports are built from clustering based on information and knowledge.
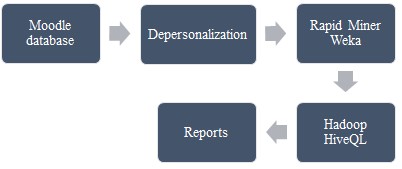
Note. The process moves from obtaining information in the Moodle database to depersonalizing. It then moves to Hadoop HiveQL for querying, and later on, it uses Rapid miner or WEKA to cluster the database (Bante & Rajeswari, 2017).
Methodology and Design
On the other hand, Canvas is an online service that facilitates learning between instructors and students through posted course-related materials. Students get communication tools and grading from professors when they submit assignments (Grossi et al., 2018). It is done electronically and boosts skill development. Colleges and universities use it worldwide to replace some of the time spent in traditional classrooms. Its features include course navigation, which links external sources, discussions, assignments, grading, and modules for reference purposes. Using the comparative quasi-experimental method to review Canvas and Moodle, it is possible to establish critical similarities and differences in big data processing.
Canvas has a modern, fresh-looking interface, while Moodle’s interface is a little bit unintuitive to work with and is not user-friendly. However, it is fully customizable, and users can change the look and feel with free and paid themes. In terms of teaching, assessing, and learning tools, Moodle is more powerful (Öztürk & Gürler, 2020). Moodle is also easy to use as it supports many authoring tools that help learners build courses from scratch. It also has suitable e-learning materials but fails when it comes to its clunky navigation and complex system.
However, Canvas is easier to navigate and has a simple design which is excellent for beginners. However, there is no control over discussion boards which can be overwhelming, and it may prove not easy to make complex uploads. Moodle offers better grading as it supports more than 12 types of assessments and uses the rubric score as the assignment score (Grossi et al., 2018). Canvas has lesser alternatives for grading, and you have to enter grades for assignments manually. However, grading forums are much simpler to use in Canvas than Moodle (Öztürk & Gürler, 2020). Both Canvas and Moodle have excellent and easy integration with third-party applications but could benefit from upgrades as they might have glitches from time to time.
Conclusion
Pricing can be a big worry to consumers, but Moodle and Canvas have free and paid versions. Moodle edges this round as it does not charge any implementation fees or set up payments like Canvas. When it comes to the target audience, Moodle is better suited than Canvas because it encompasses all schools, colleges, universities, and even corporations. Canvas’ open-source solutions work best when hosted and can be challenging to use, unlike Moodle’s server hosting, which works perfectly. In conclusion, Moodle and Canvas transmit massive information volumes daily. While both are Big Data processing systems, Moodle is the best option as it offers better control and advanced customization.
References
Akçapınar, G., & BAYAZIT, A. (2019). MoodleMiner: Data mining analysis tool for Moodle learning management system. İlköğretim Online, 406-415. Web.
Bante, P. M., & Rajeswari, K. (2017). Big data analytics using Hadoop map reduce framework and data migration process. 2017 International Conference on Computing, Communication, Control and Automation (ICCUBEA). Web.
Brumm, B. (2019). What is a database?. Beginning Oracle SQL for Oracle Database 18c, 3-7. Web.
Grossi, M. G., Elias, M. C., Chamon, C. M., & Leal, D. C. (2018). The educational potentialities of the virtual learning environments Moodle and canvas: A comparative study. International Journal of Information and Education Technology, 8(7), 514-519. Web.
Hill, P. (2017). Academic LMS market share: A view across four global regions. e-Literate. Web.
Jlassi, A., & Martineau, P. (2016). Benchmarking Hadoop performance in the cloud. Proceedings of the 6th International Conference on Cloud Computing and Services Science. Web.
Kalema, B. M., & Mokgadi, M. (2017). Developing countries organizations’ readiness for big data analytics. Problems and Perspectives in Management, 15(1), 260-270. Web.
Kennedy, J. (2020). Big data’s economic impact. Committee for Economic Development of The Conference Board. Web.
Kiran. (2020). Moodle vs canvas 2021 – Which LMS is better?. Edwiser. Web.
Kröger, J. L., Miceli, M., & Müller, F. (2021). How data can be used against people: A classification of personal data misuses. SSRN Electronic Journal. Web.
Liao, C., Squicciarini, A., & Lin, D. (2016). LAST-HDFS: Location-aware storage technique for Hadoop distributed file system. 2016 IEEE 9th International Conference on Cloud Computing (CLOUD). Web.
Lindell, J. (2020). Big data platforms and operating tools. Analytics and Big Data for Accountants. Web.
Martins, P., & Soofastaei, A. (2020). Data collection, storage, and retrieval. Data Analytics Applied to the Mining Industry, 51-74. Web.
Mills, K. A. (2019). Anticipating big data futures for qualitative researchers. Big Data for Qualitative Research, 57-60. Web.
Mogaji, E. (2018). With the integration of learning apps, what are Moodle’s prospects?. Compass: Journal of Learning and Teaching, 11(2). Web.
Muhtar, A. A. (2017). Developing online course Portal to improve teachers’ competency in creating action research (CAR) proposal using learning management system (LMS) Moodle. Journal of Physics: Conference Series, 812, 012076. Web.
Oussousa, A., Benjellouna, F., Lahcena, A. B., & Belfkih, S. (2018). Big data technologies: A survey. ScienceDirect. Web.
Periasamy, M., & Raj, P. (2016). Big data analytics: Enabling technologies and tools. Data Science and Big Data Computing, 221-243. Web.
Sharmila S, G., Amalraj A, A., & K, S. (2021). Pulling data from multiple websites to identify best deals on auction websites. International Journal on Cybernetics & Informatics, 10(2), 219-224. Web.
Öztürk, Y. E., & Gürler, İ. (2020). Evaluation of Moodle, canvas, blackboard, and open EdX. ICT-Based Assessment, Methods, and Programs in Tertiary Education, 363-382. Web.