Introduction
This paper centres on exploring customer behaviour among Chinese consumers when making hotel bookings. The researcher chose the Chinese as a specific sub-group of the global hotel market because many studies have not explored their online booking preferences, despite their growing significance in the global tourism market. Three research objectives guided this investigation. They focused on examining if there were significant differences in how the Chinese book hotels locally and internationally, finding out the effects of online hotel bookings on their buying behaviours and examining the possibility of a link between their buying behaviours and hotel pricing strategies.
Literature Review
E-commerce has become a critical force that is defining how hotels interact with their customers. The growth of this type of technological platform means that the hospitality industry has a new reality in the way it conducts its business. More importantly, the growth of e-commerce means that stakeholders in the sector have to rethink how they do business with each other and with their customers. The researcher reviewed several literatures to understand customer buying behaviours and their effects on online purchases.
The investigation showed that functional, hedonic, innovation, and aesthetic needs influenced consumer-buying behaviours. The researcher also investigated theoretical and empirical models explaining consumer behaviour in the online marketplace and established that the theory of planned behaviour, the model of goal-directed behaviour, the goal setting theory and transaction/utility frameworks shaped consumer decisions.
The above-mentioned frameworks were instrumental in identifying the main factors influencing consumer-purchasing behaviours, which were pivotal in conducting the investigation about the behaviours of Chinese consumers when making hotel bookings. These factors included consumer attitudes, people’s emotions, market mavens, buyers’ subjective norms and perceived self-efficacy. These elements of analysis emerged as the main factors influencing buying decisions. Consequently, they formed the foundation for an in-depth investigation of the main factors influencing purchasing decisions of Chinese people.
Methodology
This study is grounded in the mixed methods research approach. The model contains aspects of both qualitative and quantitative research frameworks. Relative to this fact, the researcher chose it because it provided a corroborative framework for analysing both qualitative and quantitative aspects of the research investigation. In this framework, the researcher used the findings of the qualitative research approach to corroborate those of the quantitative research method. Similarly, the findings of the quantitative research technique were used to corroborate those of the qualitative method. The concurrent triangulation technique was the selected research design. It does not contain any bias towards the collection of either the qualitative or quantitative research approaches. Therefore, the researcher collected both qualitative and quantitative data concurrently.
Data was collected using questionnaires that were administered as a survey and distributed to 50 respondents who were chosen through a purposeful sampling method from a database of clients who visited the Marriott Hotel through one of its booking agents. The data collection instrument was structured into two parts. The first one captured the respondents’ demographic information, such as age, sex, and educational background, while the second part contained their views about six dimensions relating to online booking behaviour. These dimensions included attitudes, positive emotions, negative emotions, market mavens, subjective norms, and perceived self-efficacy. The respondents were supposed to react to 24 statements relating to all of them. Their views were analysed using the SPSS technique (version 23) and subjected to descriptive and inferential analyses.
Findings and Discussion
The researcher found that all the six dimensions investigated in the study (attitudes, positive emotions, negative emotions, market mavens, subjective norms, and perceived self-efficacy) were correlated. The Pearson’s correlation coefficient affirmed this correlation because it had a significance value of 0.000 for all the dimensions explored. Although significance was established among the variables investigated, the highest correlation was found between the fourth and fifth dimensions (market mavens and subjective norms). The second highest correlation was found between the fourth (subjective norms) and first (attitudes) dimensions, while the weakest correlation was between the first (attitudes) and second (positive emotions) dimensions.
It was speculated that the above findings were influenced by demographic variables relating to the sample population. For example, the researcher deduced that most of the information or views presented in the study were largely representative of the men who formed the majority of the respondents (79%). It was also assumed that most of the views highlighted in the study were largely representative of young Chinese tourists because a majority of the respondents (42%) was under 30 years. In addition, 37% of them were between the ages of 30 and 40 years. Broadly, this finding means that about 80% of the respondents who gave their views in this study were below the age of 40.
Conclusion
The responses gathered in this study demonstrated that the effects of online booking on the buying behaviours of Chinese customers are wide and far-reaching. Particularly, the emphasis on the six dimensions investigated in this study to understand the online booking behaviours of Chinese customers showed that market mavens, subjective norms, positive emotions, negative emotions, perceived self-efficacy, and attitudes had a positive impact on the consumer booking behaviours of the target respondents.
Based on this analysis, it is possible to argue that online hotel booking has an effect on the buying behaviours of Chinese customers because it evokes positive and negative emotions on their overall purchase experiences. These views stem from the affirmative relationship between consumer buying experiences and online consumer behaviours, which were generated from the respondents’ tendency to “agree” with the 24 statements posed to them. The findings highlighted in this report are consistent with others, which suggest that customers often look out for deals that give them value for their money.
This point of view suggests that the perception of hotels and their customers regarding product value and risks are the main forces influencing pricing strategies. Based on these findings, it is assumed that the consumer behaviour of Chinese customers when making hotel bookings is part of an evolving concept, which is characterised by how different generations shop for goods and services.
Investigating Behaviour of Chinese Customers when Making Hotel Bookings
Introduction
This paper centres on understanding customer behaviour when making hotel bookings. The review is focused on examining the customer buying behaviour of Chinese customers as a specific sub-group of the global hotel market. This chapter provides an understanding of the research topic and an evaluation of the research processes that will be undertaken to support it. It is divided into six key sections. The first one explains the contents of the chapter and the second section provides a background of the topic under review. The third part of the chapter provides a rationale for choosing the research topic and the fourth section explains the modalities for undertaking the study. The aim and objectives that will guide the investigation appear in the fourth section of this chapter and they pave the way for a summary of the whole chapter, which is the sixth and final segment of the chapter. The background of the research topic appears below.
Background of the Topic
Pricing is a key part of hotel operations. Indeed, it is a significant tool used by players in the hospitality industry to increase sales and maximize revenue opportunities. It also conveys the value that they offer to their customers and indicates how much money guests are willing to pay for goods and services. Concisely, like Vimi (2013) points out, many progressive hotels should think about what customers consider before they book a hotel. While airlines and restaurants use different pricing strategies to increase demand, hotels are known to rely on a room-based pricing option that is cognizant of the prevailing demand and customer sensitivity levels in the market (Vimi 2013).
Relative to this assertion, several research studies have shown that price dispersion and differentiation are commonly practiced by several hotels that operate in the online marketing space (Ivanov 2014; Adhikari, Basu, & Raj 2013). In a research study by Ivanov (2014), it was reported that many hotels often engage in price wars. The findings of the same study revealed that, the relevance and success of hotels depend on their ability to constantly monitor the market and adjust their prices accordingly (Ivanov 2014).
Four main issues often affect the pricing strategies of hotels: customer behaviour when booking, technological advancements, modalities for booking, and independent tastes and preferences that affect pricing options (Ivanov 2014; Adhikari, Basu, & Raj 2013). The multiplicity of factors affecting existing marketing strategies shows that pricing is a complex concept that influences hotel operations (McGuire 2015). While these insights underscore how hotels choose to price their products, the focus of this dissertation will be on customer behaviour when booking. Many trends are influencing this phenomenon. Online booking is at the top of the list because experts have shown that most hotel pricing strategies adopted today are increasingly being shaped by the growth and spread of e-commerce (Bambauer-Sachse & Massera 2015).
Mobile booking trends are also gaining momentum around the world and they are increasing the intensity of engagements between hoteliers and their clients. According to Orisys Infotech (2018), mobile bookings accounted for 24% of all hotel reservations made in 2014. In 2016, this percentage increased to 30%, and in future, the trend is expected to gain more momentum (Orisys Infotech 2018).
This trend is partly buoyed by an increased appetite among young customers to book hotels via their smartphones, thereby allowing hotels to generate more bookings through this platform (Riley 2015). At the same time, there is a growing trend among customers who want real-time services through personal alerts and 24/7 support services. Broadly, these insights show a significant behavioural change in the hotel industry.
Based on the above dynamics, it is common to find many businesses in the hospitality industry investing many resources in studying online customer behaviours (Wearne & Morrison 2013). To understand how pricing strategies influence hotel operations, researchers are increasingly investigating how different contextual factors could be leveraged to maximize revenue through the application diverse pricing options (Viglia & Abrate 2014). Indeed, as Bambauer-Sachse and Massera (2015) demonstrate, if consumers are not targeted well, or hotels fail to understand the contextual factors that affect their decision to make a reservation, they would lose revenue through lost markets share. Over time, if this trend persists, it could lead to a decline in market share and possibly the failure of a business (Ivanov 2014; Adhikari, Basu, & Raj 2013).
These facts demonstrate the need to understand customer behaviour when making hotel reservations because it is a core part of developing a successful marketing program. However, most hoteliers undervalue the importance of this process.
The Rationale for Choosing Research Topic
Chinese tourists are deemed a dominant market for global tourism (Hung & Li 2016). With 130 million tourists travelling annually and spending more than $200 billion in their trips, Chinese tourists are having a significant effect on major world tourist destinations and hotels. The same demographic is also having a significant impact domestically because the World Tourism Organisation reports that they make up to 4.4 billion trips each year (Li 2015).
At the same time, they are the leading global outbound market because, in 2016, there were more than 130 million departures from China (Hung & Li 2016). Experts report that this number is set to increase in the coming years because it has been increasing by double digits since 2010 (Li 2015). Notably, Chinese tourists spend more than double the global average in accommodation costs (Li 2015). Based on these statistics, it is difficult to understand the limit of Chinese tourists, but, more importantly, it is essential to understand the consumer buying behaviours of this customer group.
This research is premised on understanding the behaviour of Chinese customers when booking hotels in and out of China. The rationale for choosing this topic is premised on the fact that the hospitality industry has not fully understood the complexity that surrounds the behaviour of Chinese customers in the global tourism industry (Hung & Li 2016). This complexity is partly explained by the rapid increase in the income of Chinese customers within the last two decades and the introduction of new marketing concepts in the Chinese market. Indeed, as Dixit (2017) points out, consumer behaviour is an evolving concept and understanding how different generations shop for goods and services could reveal different significant trends in how customers today (and in the past) book hotels.
Another issue that explains the differences in Chinese consumer behaviour is the varying levels of tastes and preferences in different Chinese regions (Hung & Li 2016). This complexity in the behaviour of Chinese customers has started to be evident globally because the socialist nation is becoming a huge market for several tourism products around the world (Dixit 2017). Therefore, the findings of this study will be useful to the hospitality industry because it will demystify the buying behaviours of Chinese customers. Lastly, they will also add to the growing body of academic literature concerning international marketing in the hotel industry.
Modalities for Undertaking the Research
This research will examine consumer behaviour related to online hotel deal purchases. It will be undertaken by consulting online travel intermediaries as the main link between the researcher and the participants. Based on research findings developed from consumer psychology reviews, the quantitative research method would provide the overarching framework for the investigation. The justification for using it lies in its efficacy in discovering the latent factors influencing hotel purchasing decisions (Reis & Judd 2014). It will also help in understanding how the purchasing behaviours investigated in this study will influence the motivation and intention of Chinese tourists to purchase hotel deals (Hair et al. 2015). This information would also be useful in understanding the demographic characteristics of clients who are likely to book hotel rooms on international trips.
For purposes of this study, information will be collected from 50 customers who will be randomly chosen from the database of online travel intermediaries of Marriott Hotel. The data collection process will be undertaken using surveys. As recommended by Saunders, Lewis, and Thornhill (2007), all the responses that will be received in the research will be anonymous and confidential. The participants will also have the option to withdraw from the study without any repercussions. The surveys will be undertaken using semi-structured questionnaires and the findings will be analysed using the SPSS method. The data analysis process will be descriptive because the nature of the current investigation is exploratory. The aims and objectives of the study appear below.
Aims and Objectives of the Study
Aim
To understand Chinese consumer behaviours towards online hotel bookings
Objectives
- To investigate if there are any significant differences in how the Chinese book hotels locally and internationally.
- To find out the effect of online hotel bookings on the buying behaviours of Chinese customers.
- To examine the possibility of a link between consumer buying behaviours and hotel pricing strategies among Chinese guests.
Conclusion
This chapter has shown that Chinese customers form an important subgroup of global tourists who are influencing the hospitality industry in different tourist destinations around the world. However, they form a complex submarket of global tourists because of their diversity. This study is premised on the need to understand their buying behaviour when booking hotels. This goal is summarised by the aim of this study, which is to understand their consumer-buying behaviour both locally and internationally. Three research objectives will guide the investigation.
They are centred on examining if there are any significant differences in how the Chinese book hotels locally and internationally, finding out the effect of online hotel bookings on the buying behaviours of Chinese customers and examining the possibility of a link between consumer buying behaviours and hotel pricing strategies among Chinese guests. The next chapter will contain a review of existing literature surrounding this research topic. Notably, it will explain the theoretical and empirical foundations of consumer buying behaviour in contemporary research studies and their contribution to the understanding of the research investigation.
Literature Review
Introduction
This chapter contains a review of existing literature concerning hotel pricing strategies and customer booking behaviours. The analysis is centred on reviewing the main factors influencing pricing in the hospitality industry and their contribution towards understanding customer behaviours in hotel booking. However, before delving into the details of this analysis, it is (first) important to understand the critical forces influencing the hospitality industry.
E-Commerce
Many studies consider e-commerce to be a critical force defining how hotels interact with their customers (Chae, Koh & Prybutok 2014; Buil, Martínez & Chernatony 2013b). The growth of this form of technological advancement means that the hospitality industry has a new reality in the way it operates. More importantly, the growth of e-commerce means that stakeholders in the sector have to rethink how they do business with each other and with their customers. Studies that have investigated how companies operate on the online platform have shown that consumer-buying behaviour is mostly influenced by functional, hedonic, innovation, and aesthetic needs (Chae, Koh & Prybutok 2014; Buil, Martínez & Chernatony 2013b).
Before the growth and spread of the internet in the hospitality industry, global distribution systems (GDS) played a key role in facilitating international commerce. According to Buil, Martínez and Chernatony (2013b), the GDS played a functional role in the industry by coordinating the functions of brick-and-mortar businesses and travel suppliers. However, with the growth and development of e-commerce in the 1990s, hotels and travel agencies changed how they operate their businesses by embracing the virtual platform (Jang, Tang & Park 2013).
At the time, major global hotels embarked on an aggressive marketing campaign to popularise their brands online (such as through their websites) (Chen 2014). A more interesting trend that emerged at the time was the reclaiming of value chain controls by hotels from travel agencies, which were their main source of business. Afiouni, Karam, and El-Hajj (2013) refer to this trend as “disintermediation.” Today, hotels and airlines have fully embraced this trend because they reach out to their customers directly to improve their business-customer experience and enhance customer satisfaction standards.
Buil, Martínez, and Chernatony (2013a) say that online direct distribution is more important for hotels that have loyalty programs than those that do not have the same programs. This is because when customers visit their websites, they are rewarded for doing so (repeat business). At the same time, loyalty programs are important to these businesses because they allow them to maintain a database for improving their products and services further (Jang, Tang & Park 2013).
Many hotels that develop individualised services commonly use this strategy (Afiouni, Karam & El-Hajj 2013). Moreover, most of them have used the same plan to forge relationships that are more intimate with their customers. Based on the success of such methods, several research studies have pointed out the need for hotels to use direct marketing platforms to build effective customer relationships, and bolster customer loyalty standards (Chae, Koh, & Prybutok 2014; Buil, Martínez & Chernatony 2013b).
Hua, Morosan, and DeFranco (2015) say that the growth and development of e-commerce have resulted in the increase of business opportunities in the hospitality industry. Online travel retailers have realised the potential that exists in this space and exploited it to account for about 50% of all online hotel bookings today (Chen 2014). Four main types of travel intermediaries have done so and they include virtual travel sites, meta-search engine sites, social media sites and flash sales sites. Online travel agencies that have relied on e-commerce for growth include Orbitz.com, Expedia.com, and Hotel.com (Chen 2014).
Other companies that have achieved the same level of success include Travelocity.com and Priceline.com. These online travel agencies operate using one of three business models: commission-based model, a merchant framework, and the opaque selling model (Chen 2014).
Meta-search websites operate differently from online travel agents because they obtain information from a list of websites and package the same data to create a common database for customers to select a hotel, based on individual preferences. Google is one of the most commonly used meta-search websites for making hotel bookings, but others that provide the same service, and have a relatively significant following, include Kayak.com and Bookingbuddy.com (Hua, Morosan & DeFranco 2015). Generally, these websites streamline the search process for their customers. In other words, they not only allow users to view available accommodation facilities but also compare them with each other.
Social media websites are different from meta-search websites because they provide user-generated data. Many hotels ignored this platform for a long time because of its unregulated nature, but have recently reconsidered this decision. Consequently, many of them run social media accounts on various platforms, such as Facebook and Twitter, to direct customer traffic to their websites and to address some of their customer issues online. TripAdvisor is one of the world’s most popular user-generated online platforms in the industry (Hua, Morosan & DeFranco 2015).
Lastly, flash sale platforms offer customers limited products for a subsidised price and for a limited time (Chiu & Chen 2014). Usually, these offers last for up to 48 hours and they are often given to customers who have registered accounts on the platforms. Some of the most common websites that fit this description include Groupon-Getaways and Living Social-Escape. Many hotels collaborate with such companies for several reasons, including increasing off-season occupancy rates and enhancing the profile of their properties (usually to appeal to new market segments) (Chae, Koh & Prybutok 2014; Buil, Martínez & Chernatony 2013b).
The Impact of E-Commerce on Hotel Pricing
Customers are often on the lookout for deals that give them value for their money. This point of view suggests that the perception of hotels and their customers regarding product value and risks are the main forces influencing pricing strategies. Studies also show that consumers often have different risk reduction strategies that they employ while shopping online because of the uncertainty associated with making virtual reservations (Hua, Morosan, & DeFranco 2015).
For example, some customers are hesitant to make reservations because of the belief that better deals will show up if they wait. Based on this analysis, many hotels are starting to realise that they are working with a group of well-informed customers who are always out to exploit suppliers’ pricing strategies. Based on the realisation that the number of tech-savvy customers is slowly rising, many hotels are under pressure from experts who suggest they should use a dynamic pricing strategy across all their distribution channels (Hua, Morosan & DeFranco 2015). However, Monga and Kaplash (2016) caution that the application of this dynamic pricing strategy requires a full realisation (by hotels) that it involves the interplay between a customer’s perceived value of the hotel’s products and the perceived risks of the hoteliers.
Several researchers have identified the dynamic pricing strategy as having the potential to increase hotel revenue because it not only attracts price-sensitive customers but also uses analytical pricing models that include different types of information, including the history of customers and their characteristics, to ascertain the right price (Monga & Kaplash 2016). This pricing model also focuses on real-time reservations, which are critical in the establishment of customer behaviour in the long run.
Online Consumer Decision-Making Processes
According to the consumer decision-making model (CDP), hotel guests often undergo three key stages before they make a decision to purchase a good or service. The first one is “need recognition,” whereby they establish why they want to make the purchase in the first place (Afiouni, Karam & El-Hajj 2013). The second stage involves searching for information, whereby they seek to find alternatives in the market that would allow them to fulfil their desired needs. The third stage of the purchasing process involves the evaluation of alternatives. The three steps of the purchasing process are considered part of a wider cognitive process that explains the decision of customers to make a purchase.
The growth of the internet has affected how the traditional CDP works because it has influenced how customers gain access to information regarding their purchasing decisions. In one study, researchers pointed out that most customers believe the internet influences their decisions to reserve hotel rooms at least 40% of the time (Riley 2015). Since the internet and technological advancements are not static, experts believe that online consumer behaviour is set to become more complex (Afiouni, Karam & El-Hajj 2013).
Different factors influence the consumer decision-making process. At the top of the list are economic issues, such as the time spent searching for the best deals online and its associated monetary costs (Riley 2015). The second issue is computing requirements, which encompass search-engine optimisation needs and the involvement of online third-party agents in hotel booking (Riley 2015). The last consideration is psychology, which influences how guests undertake their search processes. Based on the multiplicity of these factors, Chen (2014) says it is important to understand the complexity of customer purchasing decisions
Bambauer-Sachse and Massera (2015) presented a model for understanding online consumer behaviour by re-examining the traditional CDP. Using his model, the researcher demonstrated that consumer behaviour was partly influenced by external factors, including individual characteristics and social influences when making online purchasing decisions (Bambauer-Sachse & Massera 2015). Other contributors to the process include situational and economic factors that influence decision-making processes in the online environment. The diagram below shows the makeup and structure of this model.
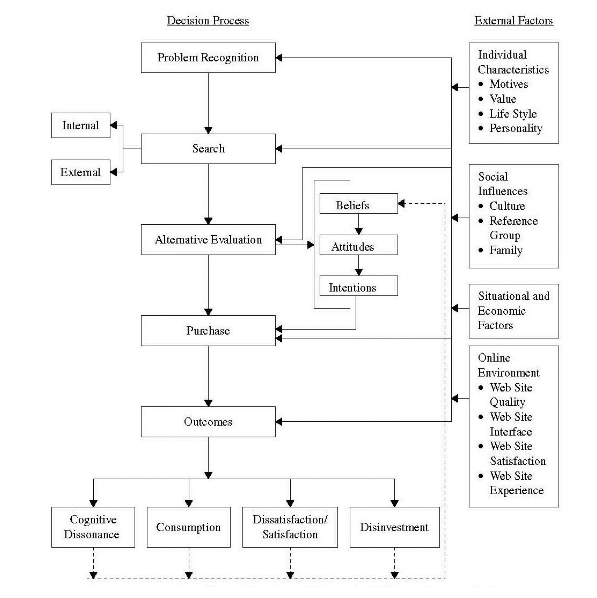
According to figure 2.1 above, processes that involve problem recognition, search-engine optimisation, alternative evaluation, purchase and outcomes guide the decision-making process. Different external factors support this decision-making process and they include individual characteristics, social influences, situational and economic factors, and the online environment. Generally, these findings show that the consumer decision-making process has evolved into a complex and dynamic system, especially when it is analysed in the context of the virtual environment.
In terms of making online purchases, Becerra, Santaló, and Silva (2013) contend that a customer’s experience and the heterogeneity of hotel products have the greatest effect. Comparatively, Becerra, Santaló, and Silva (2013) say that many consumers attach both informational and transactional aspects of consumer purchasing decisions to their online booking decisions (especially when evaluating travel products). Although most customers spend a lot of time evaluating existing travel and accommodation options, their decisions are mostly informed by informational aspects of their purchases. Notably, consumers are keen to analyse all existing information before they make their purchases online (Vimi 2013).
Evaluation and Comparison Process
Several researchers have investigated, evaluated and compared the experiences that customers undergo before making online bookings (Orisys Infotech 2018; Reis & Judd 2014). For example, Dolansky and Vandenbosch (2013) have shown that the process is subject to many individualistic and context-specific factors. Researchers who hold similar views have commonly cited the rational choice theory as an instrumental framework for identifying the right booking options to pursue (Adhikari, Basu & Raj 2013). This theory proposes that the process of evaluating consumer alternatives is premised on their ability to weigh the cost and values of all options available (Orisys Infotech 2018; Reis & Judd 2014).
The same theory postulates that consumers should use rational and logical processes when making their buying choices (Dolansky & Vandenbosch 2013). However, different observers have also pointed out that the consumer buying process is a complex one because it involves emotional cues that affect people’s perceptions of the value of products they intend to buy (Adhikari, Basu & Raj 2013).
Many researchers have contextualised these feelings as part of cognitive systems that influence the buying process (McGuire 2015; Bambauer-Sachse & Massera 2015). The same concepts have been highlighted as utilitarian and hedonic shopping values, which affect consumer-buying behaviours. They have also dominated consumer research studies by explaining how hotel guests make purchasing decisions (McGuire 2015; Bambauer-Sachse & Massera 2015).
Studies by Falk and Hagsten (2015) have investigated how utilitarian and hedonic values influence consumer perceptions through sales promotions and found that utilitarian benefits are often functional and cognitive, while hedonic values are experiential. The same researchers suggest that customers often use the two types of values when evaluating hotel bookings (Falk & Hagsten 2015). For example, promotional benefits in hotel bookings that convey utilitarian values may appeal to a selected group of customers because they provide monetary savings and increase the value of products they are getting (Falk & Hagsten 2015). Comparatively, promotional campaigns that have hedonic values have emotionally gratifying and emotional benefits.
Some researchers also argue that utilitarian values are associated with consumption utility values (Johnson & Cui 2013). News, anticipation and memory utility benefits are also associated with utilitarian values. Comparatively, consumption utility is primarily linked to the perceived value consumers get from the consumption or purchase of goods and values (Falk & Hagsten 2015). For example, it could manifest when guests book a hotel room through coupon redemption. Comparatively, news utility refers to the perceived value customers get from receiving timely and informative messages. For example, advertisements of monetary savings fit the description of this type of utility (Falk & Hagsten 2015).
Comparatively, anticipation utility refers to people’s perceived consumption value. For example, desired discounted levels fit this description. Lastly, memory utility refers to customers’ experiences, which often create assimilation effects that could be linked to their current experience of the booking process (Johnson & Cui 2013). At the centre of investigations that strive to understand consumer behaviour when making online purchases is a growing body of literature that is premised on new and existing theories which have also tried to investigate the same behaviour.
Theoretical Analysis
Transaction and Acquisition Utility Theory
Proponents of the transaction theory postulate that consumer behaviour is often influenced by several types of activities that have different consequences, depending on the type of decision made (Dixit 2017). The decision-making process is modelled around a framework, which Lee and Jang (2013b) call a “mental accounting system.” Many researchers say the framework is informed by transaction and acquisition utility systems (Dixit 2017). The transaction utility is defined by the difference between the selling and reference prices. Generally, it refers to feelings of pleasure or disappointments that customers may feel when making a deal. Thus, the value of transaction utility largely refers to the ability of customers to understand whether they are getting a good or bad deal through a purchase. Comparatively, acquisition utility refers to the economic value of a transaction.
Transaction and acquisition utility theories have been widely used to understand consumer-buying behaviours. Notably, marketers have used them to understand how different groups of consumers react to different promotional messages. The same researchers have investigated why certain groups of customers do not positively respond to different promotional messages (Hung & Li 2016).
Research studies investigating coupon usages have also adopted the two theories to understand how customers perceive purchase values and how they are prone to the deals they make (Dixit 2017). Nonetheless, researchers such as Lee and Jang (2013a) say traditional theories have failed to explain customer groups that respond well to certain promotional messages, and why they may fail to do so in others. In line with this view, Liang (2014) says customers are often influenced by specific experiences and have different perceived values of products and services when making purchasing decisions. In other words, both utilitarian and hedonic benefits are always in play.
Amaro and Duarte (2015) adopted the transaction utility theory to understand consumer-buying behaviours in the online marketplace. The theory was used to understand how customers perceive the value they would get from their online purchases and the nature of their purchase intentions. Their study showed that both monetary and non-monetary values influenced how consumers made their purchasing decisions (Amaro & Duarte 2015). In other words, the two elements were intrinsic motivators to their consumer decisions. Another theory that has been used in a similar context is the goal-setting theory.
Goal Setting Theory
The goal setting theory traces its roots in the field of human resource management, where researchers strived to understand the relationship between goal attainment and job performance (Chakrabarti, Barnes & Berthon 2014). This theory postulates that customers’ values and intentions are cognitive processes that influence their behaviour. Specifically, the theory encourages the development of goals because it helps people to realise their intended objectives and avoid undesirable outcomes (Chakrabarti, Barnes & Berthon 2014). At the same time, the satisfaction that one gets when achieving a certain goal is motivation for continuing to pursue them.
The goal setting theory has been applied in consumer psychology to understand customer-buying behaviours among different groups of consumers. Rong-Da Liang (2014) says that an online purchase is an example of a goal-oriented behaviour among customers. Although many consumers often have a specific desire to pursue a certain goal, their actions normally follow a predetermined behaviour, which ultimately contributes towards satisfying their desires. Therefore, goal setting is deemed a satisfying behaviour that motivates consumers to make specific purchasing decisions.
Theory of Planned Behaviour
The theory of planned behaviour (TPB) is linked to the goal setting theory because they both focus on the “expected” value of consumer behaviour and actions (Farah 2017). The same analogy is true for the theory of reasons action (TRA). Both models are designed to predict voluntary behaviours, which are influenced by two determinants of human behaviour: the attitudes of a consumer when influencing his/her buying decisions, and the social or subjective norms that influence the same buying decisions (Londoño-Roldan, Davies & Elms 2017). The TRA has been further modified to investigate perceived behavioural controls as another determinant of consumer buying behaviour. The TPB, which is an extension of TRA, shows how a consumer’s judgment about his/her purchasing decision may ultimately affect the entire purchasing process (Farah 2017). Generally, these insights reveal that a customer’s attitudes, subjective norms, and perceptions of behaviour control all have a profound impact on their behavioural patterns of purchasing.
Researchers have used the TPB and TRA theories to examine different social marketing concepts (relating to consumer behaviour), but most of their research has been focused on understanding how consumers buy familiar and unfamiliar products (Londoño-Roldan, Davies, & Elms 2017). Several pieces of literature have also shown the efficacy of the theory in the examination of traveller behaviours and destination choices, while others have demonstrated its usefulness in understanding how customers use coupons online and adopt e-commerce (Farah 2017). TRA and TPB theories largely help us to understand how cognitive processes inform consumer behaviours and how the same models help people to perceive the consequences of making purchasing decisions, which ultimately influence their behavioural patterns. The biggest criticisms voiced against the two theories centre on their inability to explain the main causes of the cognitive influences that affect human behaviour (Farah 2017). Nonetheless, most studies that have investigated consumer behaviour show that it is goal-oriented (Ivanov 2014). The goal-orientation process is defined by people’s quest to attain specific desires and their intention to disassociate themselves with undesirable outcomes.
Van Oest (2013) says that, in an attempt to get what consumers want, they need to trade something of value. For example, consumers have to spend long hours researching the best deals on the internet before they settle for something that they want. Although achieving a specific goal is at the centre of consumer purchasing decisions, TRA and TPB models were not inherently designed to accommodate any of these goals. Consequently, the component of goal attainment was ignored in their application. Thus, the two models have not been voiced as viable frameworks for understanding goal-oriented behaviours (Van Oest 2013). They are also weak in describing the psychological processes behind goal intentions.
Model of Goal-Directed Behaviour
Based on the weaknesses of the TRA and TPB models, some researchers developed the model of goal-oriented behaviour to understand human purchasing decisions (Li 2015). Like the TRA and TPB models, this framework strives to explain human intention and behaviours when making purchasing decisions. However, these two sets of theories differ on the premise that the model of goal-directed behaviour suggests that human intentions are hinged on a volatile desire to achieve a specific goal, while the TRA and TPB do not (Li 2015). From a psychological perspective, motivation explains an elicit intention by customers to achieve a specific goal by following a particular behaviour. Therefore, under the right conditions, a person’s motivation will be ignited, subject to his/her personal beliefs, intrinsic attitudes and emotions. Consequently, in a customer’s decision-making process, an individual’s motives will be directed towards the achievement of specific intentions. However, the need to do so has to be recognised first. This analysis shows that a specific motive is often targeted towards the achievement of a known intention. Figure 2.2 below demonstrates the main tenets of the model of goal-directed behaviour.
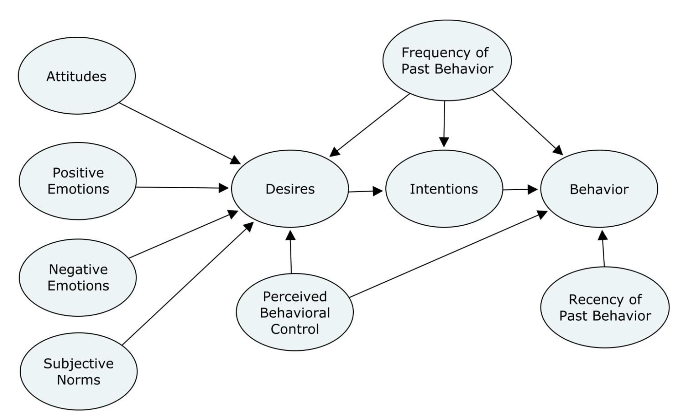
According to the model highlighted above, human desires could be explained using one of three methods: human emotions, subjective norms, and personal attitudes. Human desires play a mediating role in understanding how the three factors mentioned above influence customer purchasing behaviours. Perceived behaviour control also has a similar effect. Nonetheless, the model of directed behaviour postulates that the nature and frequency of past human behaviour significantly affect current consumer behaviours (Chen 2014).
An individual’s intentions and future behaviours are also bound to be affected in a similar fashion. The concept of “recent past behaviour” also postulates that a customer’s current behaviour will most likely shape his/her future purchases (Chen 2014). Based on the insights highlighted here, this theory provides a perfect bridge for understanding how human behaviours and intentions interact. In this regard, it accentuates the tenets of the TPB and TRA models because it provides a more powerful explanation of consumer purchasing decisions.
Wearne and Morrison (2013) investigated the role of the goal-directed model in understanding the relationship between the image of a shop and the number of customer visits it registered. The moderating variables were anticipated emotions and desires (Wearne & Morrison 2013). Additionally, the results of the investigation showed that desire and positive emotions were directly correlated to the frequency of customer visits (Wearne & Morrison 2013).
A different researcher investigated the efficacy of the model of goal-directed behaviour in understanding customer loyalty standards in a business-to-business context and found that consumer desires were largely influenced by human attitudes and positive anticipated emotions (Chen 2014). Subjective norms were seen to have the same effect as well (Chen 2014). Recent studies have also used the same theory to investigate consumer behaviour in the hospitality industry and their findings have revealed that desire is a vital impetus in the intention formation process (Van Oest 2013).
Although the model of goal-directed behaviour has been credited for expanding people’s understanding of the relationship between customer attitudes and paradigms, it has not been extensively used in consumer and marketing research. In fact, presently, few research studies investigate how the model affects purchasing decisions in the hotel industry. The current study exploits this research gap by using its salient features to explain consumer behaviour and buying intentions. The goal of using this theoretical approach is to provide a holistic framework for understanding the buying behaviour of Chinese customers in their hotel booking decisions. The conceptual framework for this investigation appears below.
Conceptual Framework
Based on the findings of this study, several pieces of literature have shown that a customer’s motivation to secure hotel deals is partly influenced by his/her attitudes, emotions, market mavens, subjective norms and perceived self-efficacy (Jang, Tang & Park 2013; Chae, Koh & Prybutok 2014). The frequency of past behaviours also moderates the relationship between customer motivation and the intention to book a hotel. Collectively, these dynamics form the main tenets of the conceptual framework, which outlines the study’s framework of analysis (Saunders, Lewis & Thornhill 2007). It appears in figure 2.3 below.
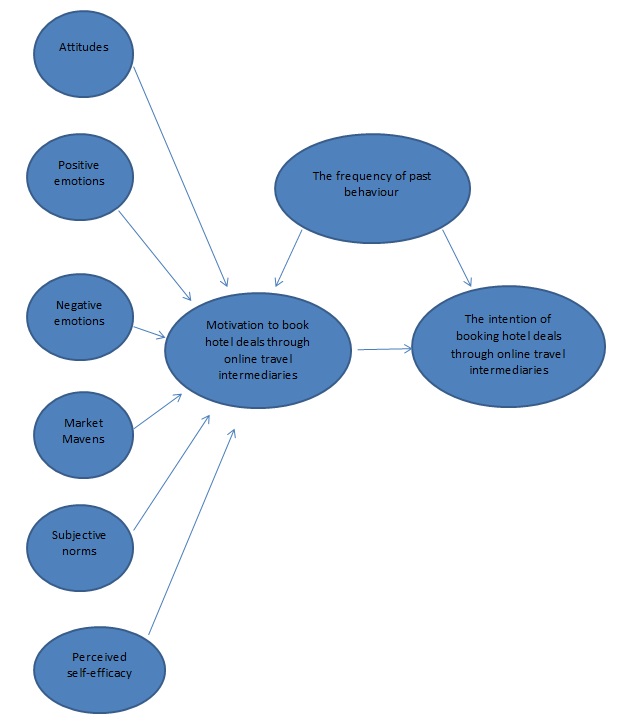
As seen from the conceptual framework above, the current study will investigate Chinese consumer behaviours by investigating how their attitudes, emotions, market mavens, subjective norms and perceived self-efficacy influence their decision to book hotels (Viglia & Abrate 2014). Past behaviours will also be used as a moderating factor to understand how an intention to reserve a hotel room will translate to a confirmed purchase. This conceptual framework is based on the findings of this literature review.
Summary
This chapter has explored the theoretical and empirical foundations of consumer behaviour in contemporary research studies. It has shown that the main models underlying this relationship are the theory of planned behaviour, the model of goal-directed behaviour, the goal setting theory and transaction and utility frameworks. These frameworks have been instrumental in highlighting the main factors influencing consumer-purchasing behaviours.
Key motivating factors that influence their actions have been highlighted in the conceptual framework and they include consumer attitudes, people’s emotions, market mavens, buyers’ subjective norms and their perceived self-efficacy. Although this literature review explains what other researchers have noted about consumer purchasing behaviour, their reviews fail to note how different customer segments respond to the same influences, subject to economic, social and political contexts. This paper fills this research gap by exploring the behaviour of Chinese consumers when making hotel bookings.
Methodology
Introduction
This chapter outlines the modalities that were followed when undertaking the proposed study. It explains different aspects of the investigation that include (but are not limited to) research technique, research design, data collection methods, data analysis techniques, and ethical considerations of the study. The first aspect of analysis is the research approach, which is explained below.
Research Approach
Two main types of research approaches are used in research – qualitative and quantitative techniques. According to Bowen, Pilkington, and Rose (2016) and Almalki (2016), the quantitative research approach is adopted when analysing measurable variables. Comparatively, researchers who are investigating subjective research issues often adopt the qualitative research approach (Olivier 2017; Saunders, Lewis & Thornhill 2007; Tracey 2013). Each technique has its unique merits and disadvantages. This study will include both techniques in a larger framework of the mixed methods research approach. The mixed methods framework will aid in evaluating qualitative and quantittative aspects of the investigation in one research study (Roberts & Povee 2014; Saunders, Lewis & Thornhill 2007). Thus, using both techniques provides the researcher will the flexibility needed to undertake an exploratory research investigation (Bowen, Pilkington & Rose 2016).
Research Design
There are six research designs applied to the mixed methods research approach. They include sequential exploratory, sequential explanatory, sequential transformative, concurrent triangulation, concurrent nested, and concurrent transformative techniques (Tobi & Kampen 2018). These research designs are briefly explained below.
Sequential Explanatory Technique – The sequential explanatory design is characterised by the use of the quantitative research approach (first) and then followed by the qualitative research technique. Researchers who want to use qualitative data to explain quantitative research findings commonly use this research design to guide their investigations (Hong et al. 2017).
Sequential Exploratory Technique – The sequential explanatory research design is characterised by the collection of qualitative findings before the use of qualitative data (Tobi & Kampen 2018). Researchers who prefer to use quantitative information to explain quantitative research findings often use it (Lucero et al. 2018). According to Bowen, Rose, and Pilkington (2017), the converse application of this research design is the sequential explanatory design described above.
Sequential Transformative Technique – The sequential transformative technique does not have any preference regarding the type of data that researchers should collect first. Instead, it integrates both types of data (qualitative and quantitative) in the interpretive section (Molina & Cameron 2014; Saunders, Lewis & Thornhill 2007). Based on the nature of this research design, researchers who are open to the use of any type of research methods, relative to how they fit within a theoretical framework, use the sequential transformative technique (Tobi & Kampen 2018). In other words, this research technique is often adopted in situations where the methods adopted are meant to serve a theoretical perspective (Tobi & Kampen 2018).
Concurrent Triangulation Technique – The concurrent triangulation technique works by providing a corroborative framework for the use of both qualitative and quantitative research approaches (Rovai, Baker & Ponton 2014). Here, either the findings of the qualitative research approach are used to corroborate the findings of the quantitative research approach or vice versa, where the findings of the quantitative research are used to corroborate those of the qualitative technique (Rovai, Baker & Ponton 2014). This way, data collection is concurrent. Researchers who want to use one method to overcome the weaknesses of another, often use this research design (Schoonenboom & Johnson 2017).
Concurrent Nested Technique – The concurrent nested technique allows researchers to use one method (either qualitative or quantitative) and have the other embedded in it (Rovai, Baker & Ponton 2014). This research design is often used in situations where a researcher tries to use one method to answer an aspect of research that is indirectly related to the main research focus. Therefore, information is sought at multiple levels (Rovai, Baker & Ponton 2014).
Concurrent Transformative Technique – The concurrent transformative technique is often used in research contexts where a theoretical perspective is sought and the investigation is reflected in the nature of the research questions (Rovai, Baker & Ponton 2014). As this explanation suggests, the purpose of this research design is to investigate one theoretical perspective from multiple approaches (Morse & Niehaus 2016).
Based on the varying characteristics of the research designs mentioned above, the concurrent triangulation technique is selected for use in this study. To recap, this technique works by providing a corroborative framework for the use of both qualitative and quantitative research approaches (Rovai, Baker & Ponton 2014). Therefore, in this study, the findings of the qualitative research approach were used to corroborate those of the quantitative research method.
Research Strategy
According to Braun and Clarke (2013), there are three main types of research strategies – exploratory, descriptive, and explanatory. Each one has its merits and demerits. Therefore, they are used in specific research contexts. For example, the exploratory research strategy is often adopted in contexts where a researcher is seeking to gain new insights regarding a research issue (Braun & Clarke 2013). Relative to this assertion, Carragher and McGaughey (2016) say it is used in situations where researchers want to assess new topics in a different light. Relative to this assertion, Creswell (2014) says that exploratory research strategies are often undertaken using three main techniques – expert interviews, surveys, and examining the existing academic literature.
The descriptive research strategy is another method that could be used to substitute the exploratory research method. It is used in situations where a researcher wants to describe a phenomenon, situation or event (Braun & Clarke 2013). The most common data collection techniques associated with this research strategy include questionnaire surveys, interviews, and secondary research analysis (Ponto 2015). A general assessment of these data collection methods shows that they are similar to those adopted in the exploratory research strategy. Lastly, the explanatory research strategy is different from both of the above-mentioned techniques because it is used in situations where researchers want to conduct a study to explain the existence of a specific phenomenon (Warfa 2016). For example, researchers have commonly used it to examine variables that underlie a specific research investigation (Willig 2013; Zea et al. 2014). The exploratory research strategy was selected for use in this study because the researcher sought new insights regarding the online booking behaviours of Chinese customers.
Data Collection
According to Paradis et al. (2016) and Cummings and Worley (2015), data could be collected using different ways, including (but not limited to) questionnaires, structured interviews, and semi-structured interviews. The information used to answer the research questions was collected using questionnaires that were administered as a survey. The research instruments were distributed virtually and the structure of the questionnaire is outlined in Appendix 1.
The first part of the questionnaire captured the respondent’s demographic information (age, sex, educational background), while the second part shows that six dimensions relating to online booking behaviour were investigated in the study. They include attitudes, positive emotions, negative emotions, market mavens, subjective norms, and perceived self-efficacy. Each dimension investigated in the questionnaire had a set of questions that are evenly distributed in number. Table 3.1 below explains the number of statements that were attributed to each dimension.
Table 3.1. Dimensions and Number of Statements.
According to Table 3.1 above, each of the six dimensions that was investigated in the study had four statements. The process of developing the questionnaires was adopted from the recommendations of Collingridge (2014) and Setia (2017) after considering the six dimensions of assessment. The guidelines used are as outlined below.
- The data collection instrument had to have simple wording to make sure that the respondents understood what was asked of them
- The questionnaire had to be easy to fill because the researcher wanted respondents to take the minimal time needed to complete the surveys as they were taking time away from their busy schedules to participate in the study
- The survey would have to be completed by all types of Chinese informants regardless of age or gender to make it as inclusive as possible
- The questionnaire had to be developed in easy language to improve the ability of the research informants to understand what was required of them
The 5-point Likert scale was used to answer each of the statements explained in appendix 1. Therefore, the varying degree of responses were distributed across five key elements, which included “strongly agree,” “agree,” neutral,” “disagree,” and “strongly disagree.” A core interval was used to record the respondents’ views about the research findings. The formula outlined below was adopted.
Score interval = (Maximum score – Minimum score) / Number of levels
= 5 – 1 / 6 = 0.8
The degree of agreement that was used to categorise the above statements is outlined in table 3.2 below.
Table 3.2. Degree of Agreement.
All positive values will signify correlation and will be indicated by (+1) values, while the lack of a correlation will be signified by negative values (-1). The analysis of the strength of correlation will be assessed using table 3.3 below, which is adapted from the works of Dominguez and Hollstein (2014).
Table 3.3. The strength of Correlation Scale.
Research Population and Sample
This section of research population and sample refers to how the research informants were recruited to participate in the study. The questionnaires mentioned earlier were distributed to 50 respondents who were chosen through a purposeful sampling method from a database of clients who visited the Marriott Hotel and used one of its booking agents. Elo et al. (2014) and Rahi (2017) describe the purposeful sampling technique as that which allows a researcher to be selective in choosing the kinds of people who will participate in the study.
The purposeful sampling method was selected for the study because the researcher needed to survey a selected group of respondents with specific characteristics. These characteristics are defined by the inclusion criteria, which is defined by the need for the participants to be to be Chinese and have used online booking to make a hotel reservation. Informants who shared these characteristics were purposefully selected from the database of Marriott’s hotel-booking website.
Permission was sought from the participants before they are enrolled to take part in the study. A call was placed to the informants to estimate their interest to take part in the study. All information pertaining to the research was communicated to the potential participants after which they were required to sign a consent form affirming their permission to take part in the study (see appendix 2). The confidence level for selecting the sample size was 0.95.
Research Variables
According to Magnusson and Maracek (2015), there are two main types of research variables, which are the dependent and independent variables. In the context of this study, the dependent variable was online booking behaviour and the independent variables are the six dimensions that appear in appendix 1 – attitude, positive emotions, negative emotions, market mavens, subjective norms, and perceived self-efficacy.
Data Analysis
The Statistical Packages for the Social Sciences (SPSS) technique was used as the main data analysis technique. This statistical assessment method was integrated in the study because it is a powerful tool for manipulating and deciphering the information collected from the participants (Rajasekar, Philominaathan & Chinnathambi 2013). Microsoft Excel was also used as a supportive data analysis tool in the study.
Validity and Reliability of Instruments
The findings of the proposed study were checked for validity using the member-check technique. Here, the opinions of the respondents were sought before the final publication of findings was completed. The purpose of doing so would be to make sure that the information depicted in the final report represented the actual views of the respondents (Sarantakos 2013). Therefore, the research informants were furnished with the final data and asked to evaluate if the same findings represented their views on the subject..
The Cronbach alpha technique was also used to test for the reliability of the research instruments. This instrument affirmed that the questions and statements appearing in the questionnaire yielded the same results if they were replaced with other similar questions. According to McKim (2014) and McKim (2017), the Cronbach alpha helps researchers to understand the underlying construct of their research instruments. Here, the construct refers to the underlying variables that are measured in the analysis.
Ethical Issues
According to Morgan (2014), Newby (2013), and Newby (2014) research studies that involve human subjects need to outline their ethical obligations to the informants. Three key ethical issues were addressed in the study. They appear below.
Informed Consent – The research respondents who participated in the study did so voluntarily. Therefore, they were not coerced or paid to participate in the study. They were also at liberty to withdraw from the research without any repercussions. All these details were outlined in the informed consent form described in appendix 2. The informants signed the form before participating in the study.
Privacy and Confidentiality – The names of the participants who participated in the study were not be revealed in the final report. In other words, the researcher presented their views anonymously. Similarly, the name of the agency that provided the list of respondents to be interviewed was also withheld for confidentiality purposes.
Data Management – The information collected in the study was stored safely on a computer and secured with a password that was only known by the researcher. After completing the research investigation, the information was destroyed.
Findings and Discussion
As mentioned in chapter 3, this chapter presents the findings of the research investigation. To recap, the aim of this study was to understand Chinese consumer behaviours towards online hotel bookings. Underpinning this aim are three research objectives, which strived to find out if there are significant differences in how Chinese guests book hotels, if online hotel bookings have an effect on the buying behaviours of Chinese customers, and if there is a link between consumer buying behaviours and hotel pricing strategies among Chinese guests. In this chapter, statistical findings relating to the research variables are provided and information relating to their associations explored. However, before investigating the relationship between the dependent and independent variables, demographic data findings relating to the research participants are highlighted below.
Demographic Findings
As highlighted in chapter 1 of this paper, 50 research participants were chosen to participate in this study. Three of them withdrew from the investigation without giving proper reasons, while one of them did not return completed questionnaires. In total, the researcher obtained 46 valid responses. Information relating to demographic data is explained below.
Gender
The first demographic variable investigated in this study was gender. Here, respondents were asked to state whether they were male or female. The male respondents emerged as the majority group (79.2%), while female participants were 20.5% of the total sample. These findings are represented in Table 4.1 below.
Table 4.1. Gender Distribution.
The pie chart below, which appears as figure 4.1, also represents the gender distribution of male and female respondents.
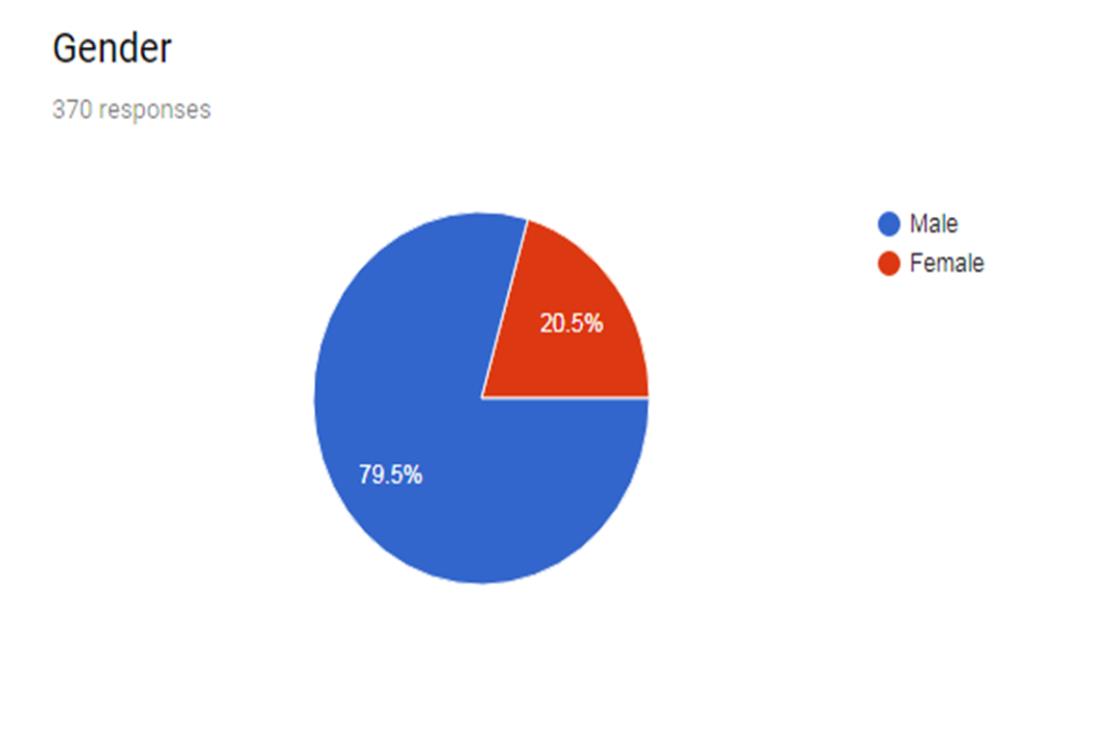
Age
The second demographic variable explored in this study was age. Here, respondents were asked to state their age group based on five key options: “Less than 30 years,” “30 to less than 40 years,” “40 to less than 50 years,” “50 to less than 60 years,” and “60 years and above.” The largest group of respondents was under the age of 30 years. They comprised 42.2% of the total sample. The second biggest group of informants was comprised of participants who were between 30 and 40 years old. They accounted for 37.6% of the total sample.
Comparatively, respondents who were between the age of 40 and 50 years comprised 13.8% of the total sample, thereby forming the third largest group of respondents. The smallest group of research participants was between the ages of 50 and 60 years. This distribution in ages could have signified a smaller representation of older guests who prefer to book hotel rooms through non-virtual means. Comparatively, the largest group of respondents was comprised of young people under the age of 30 years, signifying a heightened integration of technology in their lives. Table 4.2 below shows the distribution of the percentages across the five age groups.
Table 4.2. Age Distribution.
The pie chart below, which appears as figure 4.2 represents the same statistics in graphical format.
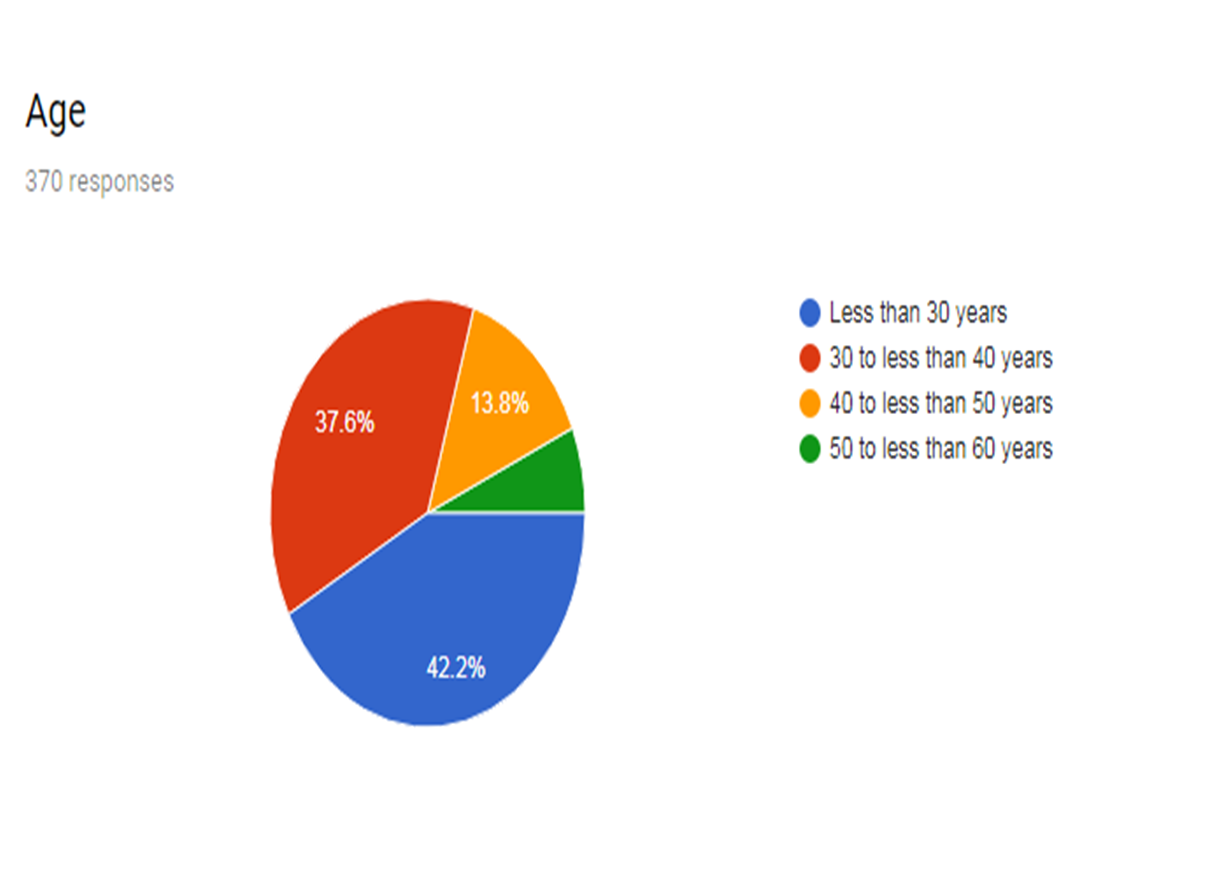
Education Levels
The third demographic variable analysed in this study was education levels. Research participants were given the option of stating their education levels across four main groups “high school of less,” “diploma,” “bachelor,” and “high school of less.” The largest group of respondents had a bachelor’s degree. They comprised 47.3% of the total sample. Respondents who polled that they had attained a “graduate studies” education level followed it. Informants who had a diploma education level comprised the third largest group of employees (17.6%), while the smallest group comprised of those who had a “high school or less” education level. This minority group comprised only 17% of the total sample. A summary of these findings is depicted in table 4.3 below.
Table 4.3. Education Levels.
The same distribution of respondents is replicated in the pie chart below, which appears as figure 4.3.
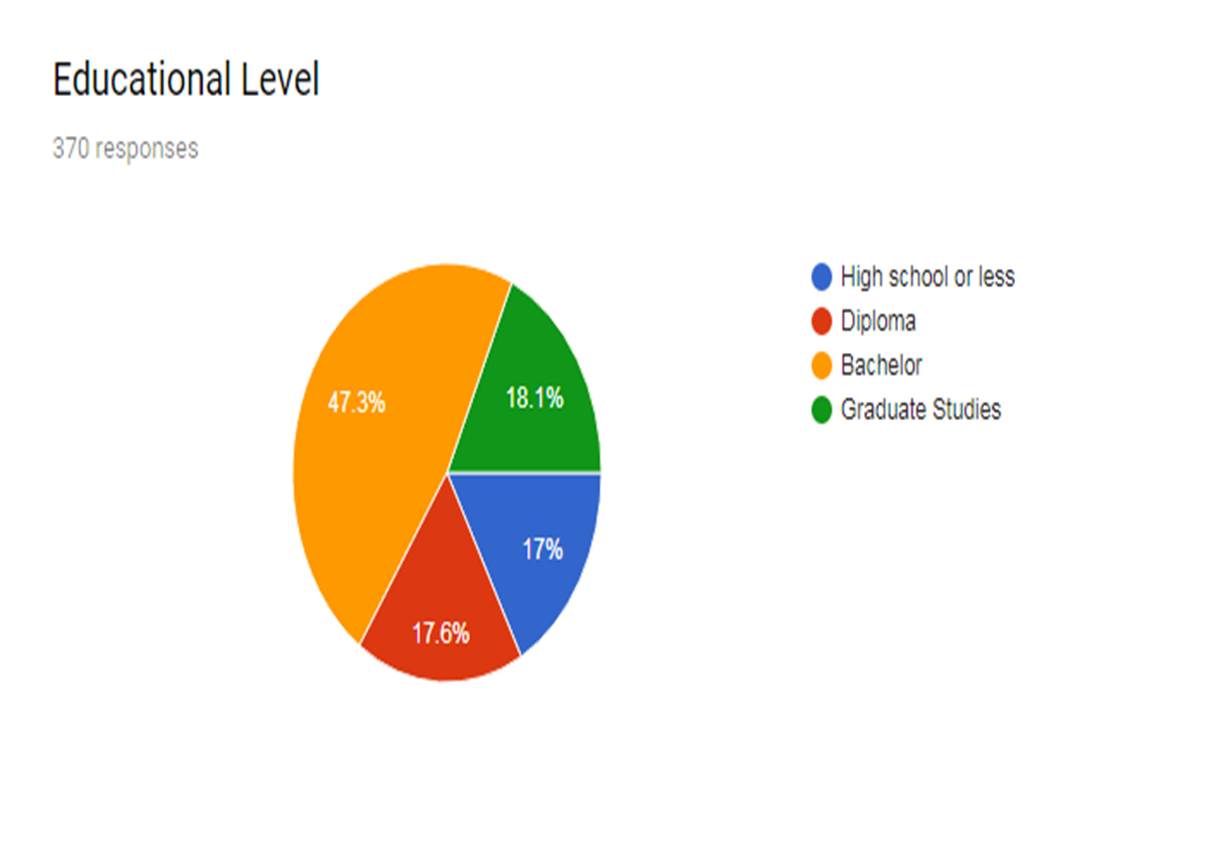
The findings mentioned above were supported by supplementary data obtained from the respondents about the research variables.
Findings of the Relationship between the Dependent and Independent Variables
As highlighted in the conceptual framework of this study, six dimensions were explored to understand the behaviour of Chinese consumers in online booking: attitudes, positive emotions, negative emotions, market mavens, perceived self-efficacy, and subjective norms. Each of these dimensions had four statements attached to them and the respondents had to answer them based on a questionnaire that rated their responses on a 5-point Likert scale. The findings appear below.
Attitudes
The findings for the first dimension (attitudes) are explored below in Table 4.4.
Table 4.4. Attitudes (1st Dimension) Findings.
According to the table above, the highest response was S3, “I trust online booking.” It had a mean of 3.275 and a standard of deviation of 1.0196. The second highest response rate was related to statement S2, which says, “I have had pleasant experiences booking online in the past.” Its mean and standard of deviation were 3.222 and 1.0385, respectively. The third highest response was S4, which says, “The services I get match what I book online.” Its mean and standard of deviation were 3.282 and 1.0853, respectively. The least (fourth) response rate was attributed to statement S1, which stated, “Online booking is fast.” Its mean and standard of deviation were 3.251 and 1.1412, respectively. The general results for this dimension of analysis are outlined below.
Result Analysis: Overall, the results derived from an analysis of the first dimension of analysis (attitudes) was 3.24 with a standard of deviation of 1.088 and an “agree” degree of response. The finding means that the informants agreed to the fact that their attitude towards online booking significantly influenced their online booking behaviour (Newby 2013; Newby 2014). It also reveals that most of the Chinese respondents were attracted to online booking because of the experiences they had when doing so. The second dimension investigated in the study related to positive emotions and its findings are highlighted in table 4.5 below.
Positive Emotions
As highlighted above, the second dimension investigated in this study related to positive emotions. The findings appear below.
Table 4.5. Positive Emotions (2nd Dimension) Findings.
According to the above findings, the highest response was observed in statement S6, which stated, “Online booking saves my time.” It had a mean of 4.112 and a standard of deviation of 1.070. The second highest response was associated with statement S5, which stated, “Booking online is convenient.” Its mean and standard of deviation were 3.261 and 1.211, respectively. The statement with the third highest response was S7, which states, “Booking online is fast.” Its mean was 3.877 and the standard of deviation was 1.125. Lastly, the statement with the least response was S8 and it stated that, “Booking online is user friendly.” Its mean was 3.192 and the standard of deviation was 1.160. The general results for this dimension of analysis are outlined below.
Result Analysis: In sum, the results depicted above show that the mean of the second dimension of analysis (positive emotions) was 3.75 with a standard of deviation of 1.135 and an “agree” degree of response. These results imply that Chinese customers are mostly attracted to online booking because of time saving advantages (Reis & Judd 2014). Convenience also significantly influenced their online booking behaviours. The third dimension investigated in the study sought to find out if negative emotions also had an effect on the online booking behaviours of the sampled customers. The findings are outlined in the section below.
Negative Emotions
As highlighted above, the third dimension investigated in the study related to the impact of negative emotions on the online booking behaviours of Chinese customers. The findings are presented in table 4.6 below.
Table 4.6. Negative Emotions (3rd Dimension) Findings.
According to the table above, the statement with the highest response was S9, which stated, “I do not get adequate assistance when booking online.” The mean and standard of deviation were 3.59 and 0.997, respectively. The second highest response rate was related to statement S10, which says, “The response time when booking online is slow.” Its mean and standard of deviation were 3.251 and 1.1412, respectively. The third highest response was S12, which says, “I have experienced incidences of theft or credit card fraud by booking online.” Its mean and standard of deviation were 3.46 and 1.028, respectively. Lastly, the statement with the least response rate was S11, which stated, “I have failed to get a refund through online booking.” Its mean and standard of deviation were 3.73 and 1.067, respectively. The general results for this dimension of analysis are outlined below.
Result Analysis: In sum, a general overview of the findings shows that the mean of the third dimension of analysis (negative emotions) was 3.7 with a standard of deviation of 1.022 and an “agree” degree of response. This result shows that the sampled group of Chinese respondents agreed to the fact that negative emotions affect their decision to book online, or not (Roberts & Povee 2014).
Particularly, the inability of the respondents to get adequate assistance when booking online was a primary cause of the negative emotions they experienced when booking online. Londoño-Roldan, Davies and Elms (2017) partly explore this effect through an investigation of negative factors that influence consumer buying behaviour. Interestingly, the inability to get refunds when booking online seemed to evoke the least negative emotions in this analysis. The fourth dimension investigated in the review sought to find out if market mavens affected the decision of the Chinese customers to book online. The results are represented below.
Market Mavens
As highlighted above, the fourth dimension investigated in the study related to the impact of market mavens on the online booking behaviours of Chinese customers. The findings are presented in table 4.7 below.
Table 4.7. Market Mavens (4th Dimension) Findings.
According to the table above, the statement with the highest response was S14, which stated, “Online booking allows me to get a wider scope of accommodation options.” Its mean and standard of deviation were 3.79 and 0.865, respectively. The second highest response rate was related to statement S13, which says, “Booking online allows me to get cheaper deals.” Its mean and standard of deviation were 3.90 and 0.895, respectively. The third highest response was S16, which says, ‘Booking online helps me to make a better and informed decision about my travel plans.” Its mean and standard of deviation were 3.39 and 1.060, respectively. Lastly, the statement with the least response rate was S15, which stated, “Booking online allows me to evaluate the kind of services offered at different establishments.” Its mean and standard of deviation were 3.43 and 1.064, respectively. The general results for this dimension of analysis (market mavens) are outlined below.
Result Analysis: In sum, a general overview of the findings shows that the mean of the fourth dimension of analysis (market mavens) was 3.55 with a standard of deviation of 1.002 and an “agree” degree of response. This result shows that the sampled group of Chinese respondents agreed to the fact that market forces affected their decision to book online (Rajasekar, Philominaathan & Chinnathambi 2013). Particularly, the possibility of getting a wider selection of accommodation options was a primary characteristic of the market, which made the respondents feel inclined to book online. Conversely, the ability of online platforms to provide an opportunity for reviewing selected accommodation plans emerged as having the least effect on their decision to book online. The fifth dimension investigated in the review sought to find out if subjective norms affected the decision of the Chinese customers to book online. The results are represented below.
Subjective Norms
As highlighted above, the fifth dimension investigated in the study related to the impact of subjective norms on the online booking behaviours of Chinese customers. The findings are presented in table 4.8 below.
Table 4.8. Subjective Norms (5th Dimension) Findings.
According to the table above, the statement with the highest response was S18, which stated, “The hotel booking site is always honest with me.” Its mean and standard of deviation were 4.23 and 0.868, respectively. The second highest response rate was related to statement S20, which says, “I believe in the information provided by the booking site.” Its mean and standard of deviation were 3.88 and 0.931, respectively. The third highest response was S17, which says, ‘I have been encouraged to book online based on other people’s experiences.” Its mean and standard of deviation were 3.93 and 0.961, respectively. Lastly, the statement with the least response rate was S19, which stated, “.The hotel booking site is genuinely concerned about its customers” Its mean and standard of deviation were 3.74 and 1.071, respectively. The general results for this dimension of analysis (subjective norms) are outlined below.
Result Analysis: In sum, a general overview of the findings shows that the mean of the fifth dimension of analysis (subjective norms) was 3.96 with a standard of deviation of 0.997 and an “agree” degree of response. This result shows that the sampled group of Chinese respondents agreed to the fact that subjective norms affected their decision to book online (Molina & Cameron 2014; Rahi 2017). Particularly, the integrity of information availed on the online platforms was a primary characteristic of the market, which made the respondents feel inclined to book online. Conversely, many respondents did not believe that the hotels were genuinely concerned about their customers. This is why this aspect of analysis emerged as having the least effect on their decision to book online. The sixth and last dimension investigated in the review sought to find out if perceived self-efficacy affected the decision of the Chinese customers to book online. The results are represented below.
Perceived Self-efficacy
As highlighted above, the sixth dimension investigated in the study related to the impact of perceived self-efficacy on the online booking behaviours of Chinese customers. The findings are presented in table 4.9 below.
Table 4.9. Perceived Self-efficacy (4th Dimension).
According to the table above, the statement with the highest response was S22, which stated, “Booking online makes me plan my trips better.” Its mean and standard of deviation were 3.79 and 0.865, respectively. The second highest response rate was related to statement S21, which says, “Booking online is a better way of making reservations.” Its mean and standard of deviation were 3.90 and 0.895, respectively. The third highest response was S24, which says, “Booking online makes me worry less about my travel plans.” Its mean and standard of deviation were 3.39 and 1.060, respectively. Lastly, the statement with the least response rate was S23, which stated, “Online booking is safer.” Its mean and standard of deviation were 3.33 and 1.064, respectively. The general results for this dimension of analysis (perceived self-efficacy) are outlined below.
Result Analysis: In sum, a general overview of the findings shows that the mean of the last dimension of analysis (perceived self-efficacy) was 3.55 with a standard of deviation of 1.002 and an “agree” degree of response. This result means that the sampled group of Chinese respondents agreed to the fact that perceived self-efficacy affected their decision to book hotels online (Sarantakos 2013). Particularly, the ability of online booking to ease travel plans was a primary characteristic of the sixth dimension analysed. Comparatively, the safety of online booking had a weak effect on the online booking behaviours of the respondents. This is why this aspect of analysis emerged as having the least effect on the analysis.
Group Statistics and Variances
Table 4.10 below explains the results of the group statistics.
Table 4.10. Group Statistics.
An analysis of the link between consumer behaviours and pricing strategies was done using the ANOVA method and the results appear in Table 4.11 below.
Table 4.11. ANOVA (effect of consumer behaviour on pricing strategies).
According to the statistics shown above, the significance value of the relationship between consumer behaviours and pricing strategies is indicated as 0.028. This figure means that the two elements are related (Morse & Niehaus 2016; McKim 2017; Rovai, Baker & Ponton 2014). The sum of squares analysis also supports the same fact, because the analysis of findings between groups was 5.600 and within groups, it was 205.190. Generally, the results of the data analysis show that the effect of consumer behaviours and pricing strategies is.028, which means that they have a strong relationship (Morgan 2014). Table 4.12 below, which was developed from ANOVA tests, also supports the same view.
Table 4.12. Consumer Behaviours and Pricing Strategies Findings (ANOVA).
An analysis of the correlation among the six dimensions highlighted in this paper revealed the following outcomes in Table 4.13 below.
Table 4.13 Correlation among Variables.
According to Table 4.13 above, all the six dimensions investigated in the paper were correlated. This fact is based on the 0.000 significance value attributed to them. They symbolize correlation because such an outcome is significant at the level 0.01 for a 2-tailed analysis (Olivier 2017). Although significance was established among the variables, the highest correlation emerged between dimensions five and four (market mavens and subjective norms). The second highest correlation was established between the fourth (subjective norms) and first (attitudes) dimensions. This is because the variables had the second highest correlation value of.749**. Lastly, two dimensions that had the weakest correlations were one (attitudes) and two (positive emotions).
The findings developed in this section of analysis have shown that all the six dimensions investigated in the study were correlated. This outcome was established because the Pearson’s correlation coefficient had a significance value of 0.000 for all the dimensions explored. Generally, this result means that positive emotions, negative emotions, risk of loss, subjective norms, attitudes, and market mavens relate with one another (Ozgur, Kleckner & Li 2015; Zea et al. 2014). However, the strongest correlations were observed between the fourth and fifth dimensions (market mavens and subjective norms). This finding aligns with the views of several research studies, which have shown the power of market dynamics and subjective norms in influencing consumer behaviours (Adhikari, Basu & Raj 2013; Chen 2014). For example, the study by Amaro and Duarte (2015) showed that the main aspects of consumer behaviour, which affected online booking activities, are artefacts, norms and shared beliefs.
Conclusion
According to the above analysis, the respondents’ views explained the effect of the relationship between the six dimensions of online booking and the online booking behaviours of Chinese customers. Relative to these findings, it is important to remember that the aim of this study was to understand Chinese consumer behaviours towards online hotel bookings. Underpinning this aim were three research objectives which strived to find out if there were significant differences in how the Chinese book hotels, if there was an effect of online hotel bookings on the buying behaviours of Chinese customers, and if there was a link between consumer buying behaviours and hotel pricing strategies among Chinese guests.
Nonetheless, based on an analysis of the demographic data, a statistically significant difference in online booking behaviours could have been caused by changes in demographic information. For example, it is possible to deduce that most of the information or views presented in the study were largely representative of the men who formed the majority of the respondents (79%). Although it could be argued that this percentage is largely biased towards portraying a male perspective of the research problem, it is also important to point out that the gender distribution reported in this study is largely representative of the gender disparities that exist in China. More importantly, it is essential to note that these disparities do not affect the quality of information presented in this review because it is an accurate reflection of the characteristics of the Chinese populace.
Based on the general age of the participants who took part in the study, it is also important to point out that the views highlighted in this study are largely representative of the younger Chinese tourists because a majority of the respondents (42%) was less than 30 years. In addition, 37% of them were between the ages of 30 years and 40 years, meaning that about 80% of the respondents who gave their views in this study were below the age of 40. Therefore, it could be argued that the findings of this review were largely representative of the opinions of a mostly young Chinese population.
At the same time, it is vital to point out that most of the views expressed in this paper are from an educated group of Chinese respondents because close to half of them who took part in the study had a bachelor’s degree. Only 17% of them had high school (or lower) education levels. These statistics imply that a majority of the employees were educated. Therefore, the pieces of information given by the respondents are mostly provided from an educated point of view.
According to the educated responses derived in this study, we find that the effects of online booking on the buying behaviours of Chinese customers are wide and far-reaching. Particularly, there was an emphasis on the six dimensions investigated in this study, which showed that market mavens, subjective norms, positive emotions, negative emotions, perceived self-efficacy, and attitudes had a positive impact on the consumer booking behaviours of the target respondents. Based on this analysis, it is possible to argue that online hotel booking has an effect on the buying behaviours of Chinese customers because it evokes positive and negative emotions on their buying experiences. The table below shows a list of the top ways in which these six dimensions affect the consumer behaviours of Chinese customers. Here, it is also important to point out that the affirmative relationship between consumer buying experiences and online consumer behaviours were generated from the “agree” response that was evident in all the six dimensions analysed. Table 4.14 below explains the top effects of online booking on the customer buying behaviours of Chinese customers.
Table 4.14. Dimensions and Top Effects.
The findings highlighted in this paper affirm the views of Buil, Martínez and Chernatony (2013a) who argued that some key characteristics of online booking would affect consumer behaviours. Other researchers, such as Farah (2017) and Liang (2014), support the same view. The findings highlighted in the table above depict the direction and ways in which different elements of virtual booking affect the booking behaviours of Chinese customers.
The findings derived in this study support the views of past researchers who have considered e-commerce to be a critical force defining how hotels interact with their customers (Buil, Martínez & Chernatony 2013b; Ponto 2015). The growth of this form of technological advancement means that the hospitality industry has a new reality in the way it operates. More importantly, the growth of e-commerce means that stakeholders in the sector have to rethink how they do business with each other and with their customers. Studies that have investigated how companies operate on the online platform have also shown that consumer-buying behaviour is mostly influenced by functional, hedonic, innovation, and aesthetic needs (Chae, Koh & Prybutok 2014).
Based on the findings highlighted in this chapter, it is also important to point out a big difference in the manner that the Chinese book hotels because they have unique values and attitudes that dictate what attracts them to virtual booking. This analysis draws attention to the six dimensions of online consumer behaviours highlighted in this chapter because unique virtues such as “honesty,” “ability to get assistance,” “time saving” and “ability to plan trips better” are important to Chinese customers. These key attributes of the target population prove that they are attracted to different merits of online booking compared to other groups of customers. For example, the study by Chakrabarti, Barnes and Berthon (2014) investigated the main driving forces behind business success and found that goal-oriented behaviours was a key driver of Chinese customers. The unique attributes of the market that attract Chinese consumers to online booking could partly stem from the cultural practices of China, which espouse values of honesty, integrity, community participation and the likes (Hung & Li 2016; Li 2015; Dixit 2017; Vimi 2013).
If these results are explored relative to the link between consumer behaviours and pricing strategies, a link between consumer behaviours and pricing strategies emerge. This link is not unique in its own right because there is a positive correlation among all the six dimensions studied. However, if the analysis is contextualized within the two variables that informed the second research objective (pricing and online booking behaviours), a link is established between the two.
The findings highlighted in this report are consistent with others, which suggest that customers often look out for deals that give them value for their money (Afiouni, Karam & El-Hajj 2013; Bambauer-Sachse & Massera 2015; Becerra, Santaló & Silva 2013; Chiu & Chen 2014; Dolansky & Vandenbosch 2013; Ivanov 2014; Johnson & Cui 2013; Lee & Jang 2013a; Lee & Jang 2013b; McGuire 2015). This point of view suggests that the perception of hotels and their customers regarding product value and risks are the main forces influencing pricing strategies.
Studies also show that consumers often have different risk reduction strategies that they employ while shopping online because of the uncertainty associated with making virtual reservations (Hua, Morosan & DeFranco 2015; Falk & Hagsten 2015; Orisys Infotech 2018; Rong-Da Liang 2014; Van Oest 2013). For example, some customers are hesitant to make reservations because of the belief that better deals will show up if they wait (Jang, Tang & Park 2013). Based on this analysis, many hotels are starting to realise that they are working with a group of well-informed customers who are always out to exploit suppliers’ pricing strategies (Riley 2015).
Based on the realisation that the number of tech-savvy customers is slowly rising, many hotels are under pressure from experts who suggest they should use a dynamic pricing plan across all their distribution channels (Hua, Morosan & DeFranco 2015; Wearne & Morrison 2013). However, Monga and Kaplash (2016) caution that the application of this dynamic pricing strategy requires a full realisation (by hotels) that it involves the interplay between a customer’s perceived value of the hotel’s products and the perceived risks of the hoteliers. Viglia and Abrate (2014) hold the same view
Lastly, although the findings of this study provide elaborate information about the online booking behaviours of Chinese customers, it is also important to point out that the same findings are only indicative. This could be one of the main limitations of the study. Based on this view, it is important to point out that future studies should use larger sample sizes, because the population sample is inadequate to reliably generalize the findings beyond an indicative framework.
Reference List
Adhikari, A, Basu, A & Raj, S. 2013. ‘Pricing of experience products under consumer heterogeneity’. International Journal of Hospitality Management, vol. 33, no. 1, pp. 6-18.
Afiouni, F, Karam, C & El-Hajj, H. 2013. ‘The HR value proposition model in the Arab Middle East: identifying the contours of an Arab Middle Eastern HR model’. International Journal of Human Resource Management, vol. 24, no. 10, pp. 1895-1932.
Almalki, S. 2016. ‘Integrating quantitative and qualitative data in mixed methods research – challenges and benefits’. Journal of Education and Learning, vol. 5, no. 3, pp. 1-10.
Amaro, S & Duarte, P. 2015. ‘An integrative model of consumers’ intentions to purchase travel online’. Tourism Management, vol. 46, no. 1, pp. 64-79.
Bambauer-Sachse, S & Massera, L 2015, ‘Interaction effects of different price claims and contextual factors on consumers’ reference price adaptation after exposure to a price promotion’. Journal of Retailing and Consumer Services, vol. 27, no. 1, pp. 63-73.
Becerra, M, Santaló, J & Silva, R 2013. ‘Being better vs. being different: differentiation, competition, and pricing strategies in the Spanish hotel industry’. Tourism Management, vol. 34, no. 1, pp. 71-79.
Bowen, P, Pilkington, A & Rose, R 2016. ‘The relationship between emotional intelligence and well-being in academic employees’. International Journal of Social Science Studies, vol. 4, no. 5, pp. 1-10.
Bowen, P, Rose, R & Pilkington, A 2017. ‘Mixed methods- theory and practice. Sequential, explanatory approach’. International Journal of Quantitative and Qualitative Research Methods, vol. 5, no. 2, pp.10-27.
Braun, V & Clarke, V 2013. Successful qualitative research: a practical guide for beginners, Sage, Thousand Oaks, CA.
Buil, I, Martínez, E & Chernatony, L 2013a, ‘Examining the role of advertising and sales promotions in brand equity creation’, Journal of Business Research, vol. 66, no. 1, pp. 115-122.
Buil, I, Martínez, E & Chernatony, L 2013b, ‘The influence of brand equity on consumer responses’, Journal of Consumer Marketing, vol. 30, no. 1, pp. 62-74.
Carragher, J & McGaughey, J 2016, ‘The effectiveness of peer mentoring in promoting a positive transition to higher education for first-year undergraduate students: a mixed methods systematic review protocol’, Systematic Reviews, vol. 5, no. 68, pp. 1-10.
Chae, H, Koh, C & Prybutok, V 2014, ‘Information technology capability and firm performance: contradictory findings and their possible causes’, MIS Quarterly, vol. 38, no. 1, pp. 305-326.
Chakrabarti, R, Barnes, B & Berthon, P 2014, ‘Goal orientation effects on behavior and performance: evidence from international sales agents in the Middle East’, International Journal of Human Resource Management, vol. 25, no. 2, pp. 317-340.
Chen, H. 2014. Consumer behavior of hotel deal bookings through online travel intermediaries. Web.
Chiu, H & Chen, C. 2014. ‘Advertising, price and hotel service quality: a signaling perspective’, Tourism Economics, vol. 20, no. 5, pp. 1013-1025.
Collingridge, D. 2014. Validating a questionnaire. Web.
Creswell, JW. 2014. Research design: qualitative, quantitative and mixed methods approaches, 4th edn, Sage Publications Ltd, London.
Cummings, TG & Worley, CG 2015, Organisation development and change, 10th edn, Cengage Learning, Stamford, CT.
Dixit, S 2017, The Routledge handbook of consumer behaviour in hospitality and tourism, Taylor & Francis, London.
Dolansky, E & Vandenbosch, M 2013, ‘Price sequences, perceived variability, and choice’, Journal of Product and Brand Management, vol. 22, no. 4, pp. 314-321.
Dominguez, S & Hollstein, B (eds) 2014, Mixed methods social networks research: design and applications, Cambridge University Press, New York, NY.
Elo, S, Kääriäinen, M, Kanste, O, Pölkki, T, Utriainen, K & Kyngäs, H 2014, ‘Qualitative content analysis: a focus on trustworthiness’, Sage Open, vol. 4, no. 1, pp. 1-10.
Falk, M & Hagsten, E 2015, ‘Modelling growth and revenue for Swedish hotel establishments’, International Journal of Hospitality Management, vol. 45, no. 1, pp. 59-68.
Farah, M 2017, ‘Application of the theory of planned behavior to customer switching intentions in the context of bank consolidations’, International Journal of Bank Marketing, vol. 35, no. 1, pp.147-172.
Hair, J, Wolfinbarger, M, Money, A, Samouel, P & Page, M 2015, Essentials of business research methods, 2nd edn, Routledge, New York, NY.
Hong, QN, Pluye, P, Bujold, M & Wassef, M 2017, ‘Convergent and sequential synthesis designs: implications for conducting and reporting systematic reviews of qualitative and quantitative evidence’, Systematic Reviews, vol. 6, no. 1, p. 61.
Hua, N, Morosan, C & DeFranco, A 2015, ‘The other side of technology adoption: examining the relationships between e-commerce expenses and hotel performance’, International Journal of Hospitality Management, vol. 45, no. 1, pp. 109-120.
Hung, K & Li, X (eds.) 2016, Chinese consumers in a new era: their travel behaviors and psychology, Routledge, London.
Ivanov, S 2014, Hotel revenue management: from theory to practice, Zangador, New York, NY.
Jang, S, Tang, C & Park, K 2013, ‘The marketing-finance interface – a new direction for tourism and hospitality management’, Tourism Economics, vol. 19, no. 5, pp. 1197-1206.
Johnson, J & Cui, A 2013, ‘To influence or not to influence: external reference price strategies in pay-what-you-want pricing’, Journal of Business Research, vol. 66, no. 2, pp. 275-281.
Lee, S & Jang, S 2013a, ‘Asymmetry of price competition in the lodging market’, Journal of Travel Research, vol. 52, no. 1, pp. 56-67.
Lee, S & Jang, S 2013b, ‘Is hiding fair? Exploring consumer resistance to unfairness in opaque pricing’, International Journal of Hospitality Management, vol. 34, no. 1, pp. 434-441.
Li, X (ed.) 2015, Chinese outbound tourism, CRC Press, New York, NY.
Liang, A 2014, ‘Exploring consumers’ bidding results based on starting price, number of bidders and promotion programs’, International Journal of Hospitality Management, vol. 37, no. 1, pp. 80-90.
Londoño-Roldan, J, Davies, K & Elms, J 2017, ‘Extending the theory of planned behavior to examine the role of anticipated negative emotions on channel intention: the case of an embarrassing product’, Journal of Retailing and Consumer Services, vol. 36, no. 1, pp. 8-20.
Lucero, J, Wallerstein, N, Duran, B, Alegria, M, Greene-Moton, E, Israel, B & White Hat, ER 2018, ‘Development of a mixed methods investigation of process and outcomes of community-based participatory research’, Journal of Mixed Methods Research, vol. 12, no. 1, pp. 55-74.
Magnusson, E & Maracek, J 2015, Doing interview-based qualitative research: a learner’s guide, Cambridge University Press, Cambridge, MA.
McGuire, K 2015, Hotel pricing in a social world: driving value in the digital economy, John Wiley & Sons, London.
McKim, CA 2014, ‘Understanding undergraduate statistical anxiety’, Journal of Research in Education, vol. 24, no. 1, pp. 204-210.
McKim, CA 2017, ‘The value of mixed methods research: a mixed methods study’, Journal of Mixed Methods Research, vol. 11, no. 2, pp. 202-222.
Molina, AF & Cameron, R 2014, ‘The acceptance of mixed methods in business and management’, International Journal of Organizational Analysis, vol. 22, no.1, pp. 14-29.
Monga, N & Kaplash, S 2016, ‘To study consumer behaviour while booking hotel through online sites’, International Journal of Research in Economics and Social Sciences, vol. 6, no. 5, pp. 158-164.
Morgan, DL 2014, Integrating qualitative and quantitative methods: a pragmatic approach, Sage Publications, Inc., Thousand Oaks, CA.
Morse, JM & Niehaus, L 2016, Mixed method design: principles and procedures, Routledge, London.
Newby, P 2013, Research methods for education, Routledge, London.
Newby, P 2014, Research methods for education, 2nd edn, Routledge, London.
Olivier, BH 2017, ‘The use of mixed-methods research to diagnose the organisational performance of a local government’, SA Journal of Industrial Psychology, vol. 43, no. 1, pp. 1-10.
Orisys Infotech 2018, Trends in the hotel & travel industry booking and consumer behavior. Web.
Ozgur, C, Kleckner, M & Li, Y 2015, ‘Selection of statistical software for solving big data problems: a guide for businesses, students, and universities’, SAGE Open, vol. 5, no. 2, pp. 1-12.
Paradis, E, O’Brien, B, Nimmon, L, Bandiera, G & Martimianakis, MA 2016, ‘Design: selection of data collection methods’, Journal of Graduate Medical Education, vol. 8, no. 2, pp. 263-264.
Ponto, J 2015, ‘Understanding and evaluating survey research’, Journal of the Advanced Practitioner in Oncology, vol. 6, no. 2, pp. 168-171.
Rahi, S 2017, ‘Research design and methods: a systematic review of research paradigms, sampling issues and instruments development’, International Journal of Economics & Management Sciences, vol. 6, no. 2, pp. 1-5.
Rajasekar, S, Philominaathan, P & Chinnathambi, V 2013, Research methodology. Web.
Reis, H & Judd, C 2014, Handbook of research methods in social and personality psychology, 2nd edn, Cambridge University Press, Cambridge, MA.
Riley, C 2015, Hotel bookings: research shows two major booking behaviour changes rising among guests. Web.
Roberts, LD & Povee, K 2014, ‘A brief measure of attitudes toward mixed methods research in psychology’, Frontiers in Psychology, vol. 5, no. 1312, pp. 1-10.
Rong-Da Liang, A 2014, ‘Exploring consumers’ bidding results based on starting price, number of bidders and promotion programs’, International Journal of Hospitality Management, vol. 37, no. 1, pp. 80-90.
Rovai, AP, Baker, JD & Ponton, MK 2014, Social science research design and statistics, Watertree Press LLC, Chesapeake, VA.
Sarantakos, S 2013, Social Research, 4th edn, Palgrave Macmillan, London.
Saunders, M, Lewis, P & Thornhill, A 2007, Research methods for business students, 4th edn, Prentice Hall, Harlow, EN.
Setia, MS 2017, ‘Methodology series module 8: designing questionnaires and clinical record forms’, Indian Journal of Dermatology, vol. 62, no. 2, pp. 130-134.
Schoonenboom, J & Johnson, RB 2017, ‘How to construct a mixed methods research design’, Journal of Sociology and Social Psychology, vol. 69, no. 2, pp. 107-131.
Tobi, H & Kampen, JK 2018, ‘Research design: the methodology for interdisciplinary research framework’, Quality & Quantity, vol. 52, no. 3, pp. 1209-1225.
Tracey, SJ 2013, Qualitative research methods: collecting evidence, crafting analysis, communicating impact, Wiley-Blackwell, Chichester.
Van Oest, R 2013, ‘Why are consumers less loss averse in internal than external reference prices’, Journal of Retailing, vol. 89, no. 1, pp. 62-71.
Viglia, G & Abrate, G 2014, ‘How social comparison influences reference price formation in a service context’, Journal of Economic Psychology, vol. 45, no. 1, pp. 168-180.
Vimi, J (ed.) 2013, Cases on consumer-centric marketing management, IGI Global, New York, NY.
Warfa, AR 2016, ‘Mixed-methods design in biology education research: approach and uses’, CBE Life Sciences Education, vol. 15, no. 4, pp. 1-5.
Wearne, N & Morrison, A, 2013, Hospitality marketing, Routledge, London.
Willig, C 2013, Introducing qualitative research: research in psychology, 3rd edn, Open University Press, Maidenhead.
Zea, MC, Aguilar-Pardo, M, Betancourt, F, Reisen, CA & Gonzales, F 2014, ‘Mixed methods research with internally displaced Colombian gay and bisexual men and transwomen’, Journal of Mixed Methods Research, vol. 8, no. 3, pp. 212-221.